The Health Pulse: AI and Bias in Healthcare
[MUSIC PLAYING] GREG HORNE: Hello, and welcome to The Health Pulse, a podcast exploring how analytics in the health and life sciences industry is growing and its repercussions in all our lives. My name is Greg, and I'm your host for this series. And as always, I'm going to be joined by my expert guests to discuss a topical subject. And last week in the episode, we were talking with Josh Morgan, and one of the things that he touched on a little bit there was this idea of equity and bias in health care. And so this week, I'm joined by a colleague, Hiwot Tesfaye, and we're going to really dig into that subject in much more detail.
But before we get started on that, as always, keep those questions and comments coming into thehealthpulsepodcast@sas.com. And as we've mentioned in previous episodes, we're going to be looking to build an episode where we start answering those questions and looking at a compilation of some of the feedback and getting all of our guests to respond to them. So without further ado, let me introduce Hiwot to you. And Hiwot, let's just start off by-- can you just tell us a bit about yourself, what it is you do and where you fit into the world of SAS?
HIWOT TESFAYE: Absolutely. I'm Hiwot Tesfaye. I currently work as a senior data scientist in SAS's Health Care Industry Solutions Team, which is a bit of a mouthful. But my day to day work is really talking to our health care customers and helping them leverage SAS technology to tackle some of their pressing health care use cases that they have. In addition to that, we're also exploring some more innovative, lightweight applications-- industry-specific applications that we can create in the health care space.
GREG HORNE: Brilliant. And one of the things we're asking all the guests at the start of the podcast is just tell us a bit about yourself that sounded way more personal or something that's-- what's Hiwot like away from the world of work?
HIWOT TESFAYE: I definitely listen to a lot of podcasts, so I'm just really excited to be on one. Let's see, outside of that, I do really enjoy doing some interesting, creative hairstyles. It's something I've been experimenting with over the last six or seven years at this point. Right now, I am rocking what we call faux locs. So they are fake dreadlocks, since I can't commit to dreadlocks right now. They're very long and it took about seven hours to do. So that's something I've been turning towards as an artistic self-expression as well of self-care.
GREG HORNE: That's fantastic. And I get to see and be with you on a fairly regular basis, so I see this in real life. And it does look fantastic.
HIWOT TESFAYE: Appreciate it.
GREG HORNE: Yeah. So we're going to look at now a bit about bias. And what I want to talk to you a bit about to start with, we talked with Josh last week about equity, as I mentioned at the beginning, and we talked about access to care. So when you think about algorithms and access to care, especially as we start changing where our front door to health care is, how do you see the dangers in what we're doing coming forward and maybe some solutions in that?
HIWOT TESFAYE: Yeah, I think it's important to first recognize that algorithms are being used across the board, not just to determine who should get access to health care or insurance or what the premiums should be but everything along the lines of reducing costs, improving health care outcomes, improving patient experience. Algorithms have some place to play in that.
But a lot of the use cases that I come across in health care have a lot to do with assessing risk, so categorizing people based on some type of risk, whether it's the risk of not showing up to a doctor's appointment-- the risk that people won't adhere to their prescribed medication or some other intervention, the risk they may develop a chronic illness the following year.
So there's a lot of risk mitigation use cases that we come across that are primed for artificial intelligence and algorithms to learn from historical data, learn the patterns of people's behavior, as well as how diseases progress and be able to predict what the outcome is going to be.
And I do think there's great promise in these types of use cases and being able to direct care based on somebody's risk score, but there's also a lot of pitfalls that we need to be aware of. And a lot of that, I think, comes from potentially not understanding how the health care system has worked historically and how different populations interact with health care systems that we, as algorithm designers, data scientists, machine learning engineers, we don't walk in those shoes of those people.
So we might not know how they interact with the health care system. So as we're designing our algorithms, we might fall victim to not being able to design it for everybody. And so the accuracy of health care algorithms needs to be, I think, of the most important, not at a global level but at the individual subpopulation level as well, to make sure that when we are creating these predictions, they are accurate for everybody across the board.
GREG HORNE: That sounds really interesting, Hiwot. And as you describe it, I'm thinking about how that makes sense and how that's going to play out in the real world. But I think to help people listening to this today, when you think about what you've just described, how might somebody experience that in their life? What as an example of an algorithm bias in health care today or that we might see play out into somebody, and how might that adversely affect their health care?
HIWOT TESFAYE: I think the most famous example that we've come across is the work of Ziad Obermeyer and his colleagues over the last-- I think it came out back in 2019 at the end of the year, where they found racial bias in an algorithm that's widely used across various health care systems in the United States. And that algorithm is really designed to predict what the health care cost of a patient is going to be in the following year.
And part of the reason they were using cost is because of this assumption that if people are heavily utilizing health care services, they are at greater health care need. Therefore, they're sicker. And so they are equating costs to health care need in this case. So what ended up happening is that even though the researchers or the algorithm designers did not include race as an input into that model, the algorithm still learned to assign a higher risk score to white patients over sicker Black patients.
And the way that algorithm was being used in the health care system was basically to prioritize patients into care management programs. So sicker, quote unquote, "sicker" or costlier patients in the following year would be admitted into care management program where they have access to additional health care services, like a nurse calling you up to make sure you're taking your medication or home visits, so additional care that's being given to those patients that have been deemed high risk by this algorithm.
And so part of the reason why the racial bias was coming through is that the algorithm designers did not account for the fact that the patterns of health care service utilization could be different by race, where for a given level of disease burden, you'll see less dollars being spent on Black patients than on white patients in the United States for a myriad of reasons-- historical and otherwise.
So the bottom line is health care costs does not equal health care need in the context of Black patients. And if that is not taken into consideration when we're designing the algorithm, it will inevitably restrict access to care for Black patients. And this is after the patients have actually crossed out front door threshold, and they're actually within the health care system and they're being driven in one direction or another based on outputs that these algorithms are providing.
GREG HORNE: OK, and you make this sound like it's a very modern issue-- this biased piece is something-- because we have AI and because we have technology, this has become a modern issue. I was just reading this morning a bit about this idea that medical devices may inherently be biased as well and the simple pulse oximeter. Are you able to talk about, historically, then is this a new problem, or is this something that we have faced for a while and we're just waking up to it? And can you just explain a little bit about some of the more positive progress in this space.
HIWOT TESFAYE: Yeah, I don't think this is a new problem by any means. I think, more than anything else, these algorithms and the mistakes that they've made and the headlines that we've seen are more of a reflection. It's a mirror that's reflecting back society and the way society works.
And I think it's interesting and it's great that people are taking a lot more interest in this topic because it's easier to interrogate an algorithm than it is to interrogate an entire health care system, and to ask the algorithm, oh, why did you give a higher risk score for a particular patient over another, even though their metrics might be identical?
So it's certainly not a new phenomenon by any means. It's just that algorithms, I think, are exposing some of the health care practices that we've had in the past and putting them at the forefront where we can interrogate it without actual, maybe, fear that somebody is going to become defensive if that makes sense.
GREG HORNE: That makes perfect sense. Absolutely, because I think one of the things in medicine is it's very much a hierarchical process, and it's a decision process. So if you think in many ways, it should lend itself to this kind of algorithm very easily. But when you start to see this bias coming into the story, I can see why that can create its own problems. So if we think about that and you think about some of the ways we can benefit, so what's the one thing you wish that people would understand about responsible AI today and how it might be delivered?
HIWOT TESFAYE: Yeah, I think it's important to understand that we need to lay down our ego a little bit and dig deeper into our curiosity, because as people in the space of data science and designing artificial intelligence systems, a lot of us come from very similar backgrounds-- similar education level. We come from a very small portion of the population, and our tools have the power to affect the course of people's lives at a much greater scale.
And so if we are able to harness this sense of empathy that we may not know how our algorithms are actually going to behave in the real world, it could go a long way. So the example that I gave you about the algorithm that was assigning people to care management programs, just a simple understanding that care service utilization could be different across racial groups could have mitigated this entire problem from the beginning.
And so I just want to make sure that people understand that we, as data scientists and people in tech, have the power in our hands to choose what parts of society we want to amplify in the world through our tools and what parts that we don't want our tools to learn and to amplify into the world. So I think there's a great deal of responsibility we should feel to ensure that we're not perpetuating historical biases that have been going on for centuries at this point and to really be aware that our tools have the power to solidify and calcify these systemic issues even further.
GREG HORNE: That's really interesting you bring that up. Now you've talked a bit about racial bias in this as well, but I want to think about other types of bias that exist too. And one of the things that I hear regularly is our aging population drives huge cost changes in health care. Now that's a bias because there are a lot of people, who are very old, who are very healthy, and there are a lot of people, who are very young, who are very healthy. Can you just reflect on some of these other biases and just talk a little bit about why they might have an impact on health systems and, maybe, think a bit about what we can do to improve that?
HIWOT TESFAYE: I think part of the reason these biases even show up, whether they're age bias or racial bias or gender bias or whatever you want to call it-- socioeconomic bias, because I'm sure a lot of it is masked by that too-- is our lack of understanding, in my opinion, of how people interact with the health care system, what their real needs may be.
I think you've given a couple examples, Greg, in the past for how the aging population doesn't always have a huge burden on the health care system. And that's just an assumption that we have in our heads that we then enact programs around, but that doesn't necessarily have to be true.
And I think we need to-- it goes back to what I was saying earlier about honing in our curiosity to ask the questions to challenge our own assumptions when we're creating these algorithms, so that the people who have either historically been marginalized or people who are vulnerable are at greater risk of being harmed by these types of decisions are taken into consideration when we're designing this. And this really starts from the very beginning of asking the question of, should we even build this algorithm? Is it appropriate in this context to try to automate this process or not?
And then from that point forward, if we say the answer is yes, at every step of the analytics lifecycle, we need to be asking ourselves questions. Is the data representative of the population we're trying to affect change in? Is the target variable distributed equally across various groups-- the aging population, as well as men and women, as well as different racial groups, and so on? And we need to continue to interrogate the data and the question that we're trying to solve at every step of the analytics lifecycle, all the way until we deploy the solution and we're training people on how to interpret their results and take action on it.
GREG HORNE: And I know one area that you've shown some interest in as well is in medical imaging. And to me, I would look at this and go, how on Earth do you get an algorithm to be biased in a medical image, because the image doesn't necessarily know how old it is, or the race of the image? All the things you've explained so far would not apply to an image, so tell me a bit about imaging and where we can fall into pitfalls there.
HIWOT TESFAYE: Yeah, I think a really interesting and probably famous example is where an algorithm was trained to try to differentiate between-- again, this is not a health care specific example, it just goes to show what I'm trying to say-- to tell the difference between a wolf and a dog, and the accuracy was great. This algorithm was really, really good at telling the difference between a wolf and a dog.
But then what they found out later on when they tried to interrogate what parts of this image of a wolf is the algorithm be able to with high accuracy say, this is what differentiates a wolf versus a dog. And what they found was that the algorithm was really focusing on the background. So a lot of images of wolves end up being taken around snow.
And so the algorithm picked up on that pattern that there's a lot of snow every time an image is labeled as a wolf. And so the algorithm was able to quickly differentiate between a wolf and a dog. Where, in reality, if we take away that background image of snow, we don't really know how the algorithm would be accurate in predicting what the image is.
So I think the example I'm trying to give here is to illustrate that the algorithms can pick up on patterns that we don't intend to train them on, and that's an important point. So even if you're not including information that you want your algorithm to learn, it still has the ability to pick up on those things, those unintended things that you are not actively telling it to pick up on.
So I think it just goes to show that we, as just human beings, have blind spots. And sometimes the algorithm can pick up on patterns that we never even imagined were there and just put it in front of us. So we just need to be constantly questioning our own blind spots, including diverse perspectives, as we are creating these algorithms to make sure that we are filling in those blind spots as we go.
GREG HORNE: OK, that's really interesting because I think people don't recognize that. So in the whole picture, then, when you think about it, do you see algorithms putting my doctor out of business? Can you see that I am going to go and see a computer who's going to diagnose me and treat me in a better way than my doctor?
HIWOT TESFAYE: Not anytime soon, in my opinion. I think AI sometimes is given a lot more credit than it deserves, not to say that it's not powerful, it is. But I think it always has to be in tandem with human decisions. It has to augment the human decision, rather than replace people as well-trained and as deeply trained as physicians are.
So I don't see that happening any time soon truly. But I do see-- we've talked about risk scores in health care, where an algorithm's decision could potentially sway how we decide to treat particular patients. And I think that is a real risk. As we're augmenting the human decisions through algorithms, if the algorithm is not accurate, it could have the power to sway what the end decision maker decides to do.
GREG HORNE: So bearing that in mind, then, who should be accountable? Where does responsibility lie? So if my doctor uses an algorithm to make a decision and it goes wrong and, maybe, my life is put at risk, or maybe I have a completely life-changing event, where does the liability lie?
HIWOT TESFAYE: That's a great question. It certainly should not solely fall on the shoulders of the lone statistician or the lone data scientist. And I'm not just saying this because I'm part of that community, but really every single person and institution that was involved in producing the data, in cleaning the data, in building the models, in deploying those models, and making decisions on those models shares part of that responsibility. And there's a lot of it to go around, in my opinion.
So I say this because every step of the analytics lifecycle is an opportunity, again, to question the data and the objectives of the project and ask ourselves questions like, is there accurate representation in the data? Are we even asking the right question here? Is there fairness and accountability baked into the entire process?
Do the people at the end of this model, the people who are actually taking the end science and making decisions on them, are they well trained on the limitations and the caveats of the tools that they're using, because they shouldn't really take the information and take it for truth or for full value. They really need to be able to understand the limitations of the tools that they're interacting with. So it's really every person that's part of this chain needs to take responsibility.
And in addition to that, I was actually listening to another podcast where Dr. Ruha Benjamin was talking about how as consumers of algorithms, so as the patient, as the student in a medical system, we need to advocate for better tools and take some responsibility ourselves. And obviously, a lot of that response should not fall on end consumers, but we should demand better of the people that are designing these algorithms, instead of just taking it as a fact as something that we can't do anything about.
GREG HORNE: I mean, it's an interesting area of liability. And one of the questions I always throw to people is I didn't believe or see a self-driving, fully autonomous vehicle in our lifetimes, not because the technology doesn't exist but more because we don't know where to lay the liability that goes with it. So bearing that in mind, just thinking about how people get confidence in that system, do you think the average person cares where the liability sits? And do you think some of the people are going to become more concerned with it as more of these algorithms come to pass?
HIWOT TESFAYE: I think that's a great question. I think it's important to recognize that we are already interacting with algorithms on a day to day basis, and a lot of us are not complaining about it. Yeah, every time I plug in the address to go to a different location in my Google Maps, that's me interacting with an algorithm. Or every time I log onto Facebook and an ad pops up, that's me, again, interacting with an algorithm.
I think there's growing awareness of the pitfalls of these algorithms now more so than ever. There was a film called Coded Bias that came out not too long ago where they featured Joy Buolamwini's and her work on facial recognition technology. There was another documentary on Netflix that came out, The Social Dilemma, I don't know if you've seen that one.
And again, I think having these kinds of documentaries and content out there that raises awareness, that with all of the amazing things that comes with having algorithms out there tailoring their recommendations specifically for you and making you feel like they really understand and see you, there's a lot of benefit to that. But at the same time, I think there is a growing awareness of the pitfalls of these algorithms.
And part of ongoing conversations around what legislation could look like in this area of algorithmic fairness, and accountability, and transparency, and so on is giving people the ability to provide feedback to the system to say this was not accurate, or to provide input back to the system that their experience with this algorithm was terrible and this is the impact that it had on their lives. So I think being able to incorporate that end user feedback back into the algorithm and inform how it's designed and made better could be something interesting that comes to light later on.
GREG HORNE: Brilliant. That's really interesting. Thanks very much, Hiwot. I'm just throwing out a question at the end of this piece. This is the last question I'm going to ask you. Think into the future, if you can imagine where we're going, what kind of things do you think we might see in the world of AI and overcoming bias in this space if you were going to really throw the ball out there and think of something that's maybe out of the listener's thought or where we might already be thinking we're heading?
HIWOT TESFAYE: I think it's important to note, for our audience, that there is AI legislation coming soon, particularly from the European Union, that is slotted to-- they're, apparently, planning to create a legislation proposal at the beginning of this year. So with that, I think there's a lot of changes that we can look forward to where a lot of accountability will be taken by software vendors as well as people that are developing these algorithms.
I do also see-- can predict, I guess, the growth of algorithmic auditing as being a new frontier of jobs that are coming out, where people are-- they need help with assessing the risks associated with the algorithms that they're designing and to figure out ways to mitigate that risk. So I do see that next frontier for people in the data science and statistics areas that they can look forward to is this algorithmic auditors type job.
GREG HORNE: Hey, Hiwot, I know I said that was the last question, well, you just got me thinking and there's something else I want to ask you about. There was a paper published recently that talked about how bias has been written into certain algorithms quite deliberately. And I wanted to see if you were aware of that paper, if you've seen it, and just some opinions of that paper and what's in there.
HIWOT TESFAYE: Yeah, I'm really glad you asked me about that. The paper is called "Hidden in Plain Sight." And it lists out 13-- I think it was around 13 different algorithms that determine what kind of treatment people should receive. And again, this is once they've crossed the threshold of the front door of health care, they're in the system, and people are trying to determine what care pathway they need to go down.
So to give you an example that's referenced in the paper-- and this is from the area of cardiology-- is the American Heart Association's Get with the Guidelines Heart Failure Risk Score, which predicts the risk of death in patients that are admitted into the hospital. So all else held equal, it assigns an additional three points for any patient that's identified as non-Black.
So those that are considered non-Black are considered at greater risk of death once admitted into hospital and then are given additional services to mitigate that risk essentially. So conversely, those that are identified as Black have three less points in that risk score. And so the ramifications of not identifying the risk in that patient population could be literally life and death.
And there's another algorithm that they mention within cardiology, again, but it's a mirror opposite of the one that I just talked about. And this one, I believe, was created by the Society of Thoracic Surgeons that estimates, again, the risk of death. But in this case, it's during surgery, so complications during surgery. And the algorithm uses race and ethnicity here as well. But in this case, all other variables held equal, patients identified as Black are given a higher risk score, when in the other one they were given a lower risk score.
So if this type of algorithm is used in a preoperative setting, you can imagine that risk score can be used as a reason not to provide that kind of operation service to patients with high risk scores. So in both cases, resources are being diverted away from Black patients because of these algorithms. And it's really interesting how in a lot of use cases where race and other demographic information are available, there's a lot of hesitations about whether we need to use them in the algorithm or not.
But in this case and the other 13 algorithms that are listed in this research paper, they just throw it all in and are using it in practice, which is a little wild. And it's not to say that these algorithms need to be abandoned immediately. I don't think I'd advocate for that without proper interrogation of how these algorithms came to be, what is the research that's backing this. I think we really need to ask those questions, and we need to ask them fairly quickly. But I thought it was just such an eye-opening paper that I hadn't come across before.
GREG HORNE: What I found interesting about it was when the authors of the algorithms were asked why they included a racial piece in there, it seemed to be a collective shrug. It's like nobody had even thought about why they'd included it. And I guess from a logic point of view, you'd probably look at it and say this is a relevant piece because we know family history, for example, is a very good indicator of health outcomes. But how do you reconcile that? Is it because you're looking at family history, or is it that you're looking at ethnicity? Can you differentiate between the two?
HIWOT TESFAYE: I think one of the authors of the paper had made a comment elsewhere about how when they're in medical school as doctors or training to become physicians, there's a lot of mention of how race is a factor or is a risk for hypertension and other various things. But we know through our social studies classes that race is a social construct and not a biological phenomenon, because those of us that are considered Black, it's such a huge category of people.
And there's a real chance that somebody who is considered Black in the United States might be more genetically similar to somebody from Europe than somebody, like me, who's from Africa. But we're all in this massive category of Black, which might not be a good indicator for biological or genetic similarities within that group for us to determine care that should be provided to that massive group of people if that makes sense.
But I think for the longest time in health care, it has just not been questioned. Race has been used as a reason for many things-- many sinister things, certainly-- and it just has not been questioned for a long time. But I think it's great that people are starting to look at these old practices and say, hey, we need to have real justification, research-backed justification to include race as a factor in algorithms that are being used in clinical settings.
GREG HORNE: Brilliant. Well, thank you very much, Hiwot. That's been a really interesting, insightful piece. And I'm sure our listeners will have lots of feedback on this as well. And just to remind you, you can do that through our email address, thehealthpulsepodcast@sas.com.
I really like this idea at the end there of algorithmic auditors. So please, we welcome comments on that and questions on that because the way the work is changing and the roles that are expanding in this space is something that we see a lot of across all our industries but particularly in health care. So I think that subject of understanding and being able to review your algorithm, using an audit path, and seeing how that applies to legislation is really interesting. And I'm sure you've got an opinion on that as our audience.
As mentioned before, we're going to bring those questions and comments in the episode where we're going to sum up and look at our discussions over the course of this series. But I just want to say thank you to Hiwot for taking the time today to be on our podcast and joining us. Thank you for joining me on The Health Pulse. I've been your host, Greg Horne. Like and subscribe to see future episodes, and we'll be back with you soon. Thank you.
[MUSIC PLAYING]
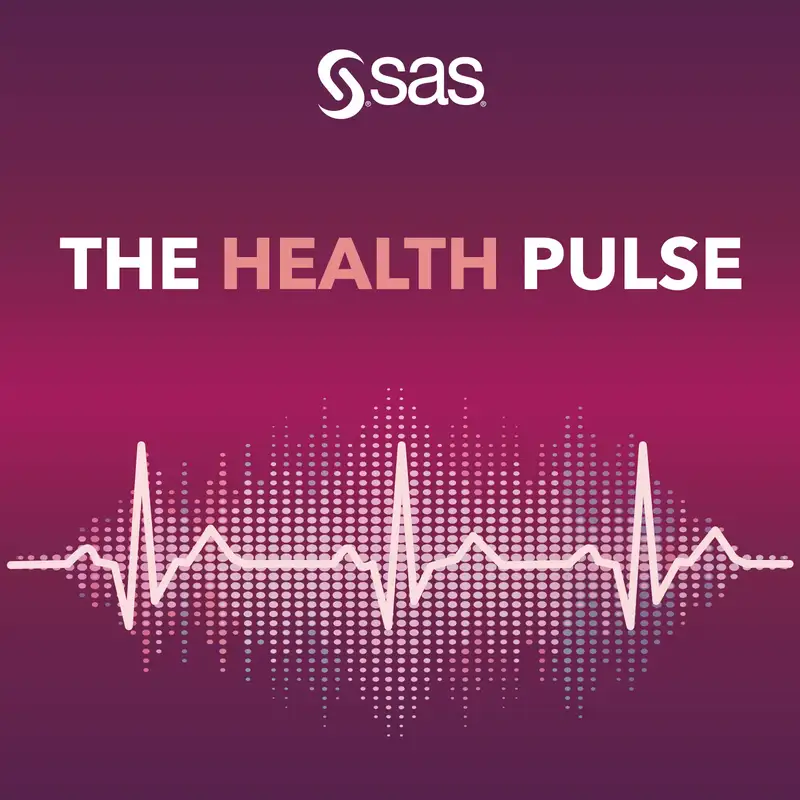