The Health Pulse S2E5: Creating a Healthier World with Ethical AI
GREG HORN: Hello, and welcome to another episode in a brand new season of the Health Post Podcast. I am your host, Greg Horn. And in season two of our podcast series we're focused on health care innovation. And looking to uncover where technology and new approaches will change the world of health care and life sciences.
As you know, we are now producing the podcast in two formats. So if you've been an audio listener in the past, then I would like to suggest you also check us out on the soft-- SAS Software channel on YouTube. And of course, we welcome your questions and comments, as always. On both that YouTube channel and also at our email address, which is thehealthpostpodcast@sas.com.
So this episode, I am going to be joined by my guest, Reggie Townsend. And Reggie is a SAS colleague. And he is the director of the newly formed data ethics practice that we've just put together at SAS, recently. And we wanted to bring Reggie back on-- bring Reggie onto this podcast, because we had some excellent feedback from an episode we did at the start of the year.
Where we talked about data ethics, and the role of analytics, and the like in bias and ethics. And that can be found on our back catalog. The interest was huge. And it just shows you that feedback really does help us with the content creation. So remember do supply comments, do email us, and help us keep adding new content to the podcast that is relevant to the audience.
So over to you now then, Reggie. So please introduce yourself. Actually, before we get into it, where about's in the world are you today?
REGGIE TOWNSEND: So I'm in Chicago-land, today. Where it is actually getting cold, I think we're supposed to get a warming trend here this weekend. Which I am very much looking forward to, because you know cold is cold.
GREG HORN: Yeah. It was 0 degrees when I got up this morning, here in Toronto.
REGGIE TOWNSEND: Ouch.
GREG HORN: Winter is very much on its way, for sure.
REGGIE TOWNSEND: I will stop complaining, right now.
[LAUGHTER]
GREG HORN: So Reggie, let's just off-- tell us a bit about your background, and the things you did prior to this role, and then a little bit about the new role.
REGGIE TOWNSEND: Yeah. So, you know, I'll spare the long drawn out version of life before SAS. Just suffice to say, I spent a lot of time in technology. A couple of decades now, which either makes me very old or experienced. You take your pick. And yeah, came to SAS a little over six years ago, Greg.
And start off doing work, from professional services standpoint, working with our life sciences organizations. From just kind of a SAS deployment perspective, right. Making sure that we were crafting the right kinds of deals for our customers. Making sure that they were getting the right technology at the right time and the right places.
Making sure that we had people showing up doing what they were supposed to do. And then got very much into our cloud work and spent a lot of time there. Just kind of joining the professional services, in addition, to our cloud activities together.
And again, it's all about developing opportunities and making sure that we were positioning the best possible solutions for the problems that we understood.
GREG HORN: Fantastic. And the other thing we always get folks to do, at the start of the podcast, is tell us about something personal. Like a hobby or an interest, what do you do when you're not at SAS?
[LAUGHTER]
REGGIE TOWNSEND: So a few things. So one that's kind of top of mine, because tomorrow is a pretty special day. So I do improv comedy, people don't know that. And so, yes. I see your face. So tomorrow, we will hit the stage for the first time since the pandemic began. So we perform with a mask on and all that good stuff. So it'll be interesting, to say the least. But yeah, I've been doing that for a number of years now. And it kind of started off with the idea of just stretching out, doing something new, just kind of getting outside of myself.
Turned out that some people said, hey you're actually decent and you know we want to have be a part of our team and the whole thing. And so, yeah. We've been performing for a number of years now together. And like I said, get a chance to hit the stage as a group again, tomorrow. For the first time in a long time. So I'm looking forward to that.
GREG HORN: That's fantastic. I love the idea of improv comedy. I've been to see a few shows like that. So yeah, that's quite something. So it would then seem to me, I could pretty much throw any question at you today and there's going to be an improvised response immediately. So that opens a challenge, I'm sure.
[LAUGHTER]
REGGIE TOWNSEND: That's fair. There's probably some truth to that. Yeah.
[LAUGHTER]
GREG HORN: So Reggie, you were recently asked to lead the creation of this new ethics practice within SAS. Can you tell me a little bit about that background? OK. And why do we need that in the first place?
REGGIE TOWNSEND: Mm-hmm. Yeah. Good question. So I like to start these kinds of responses off with a grounding, which is to say you know we've been around for 45 years, right. So it's not to suggest that we've been unethical for 45 years, right. Just because we just-- we started a practice. What we saw was that there's an increasing market need for this space.
And we'll talk, I'm sure, a little bit more about what data ethics is and what it means. But we want it to be a lot more intentional than we. You know we do a lot, in terms of technology development, deployment, and so on. And so while it is important that every individual who is involved is doing the right thing, and staying compliant with law, and all these sorts of things. All that's very important.
What you find in this space of AI, in particular, is that AI grows on itself. And so we've got to-- you need only look to the marketplace and see a number of examples where people are like legitimately getting in trouble for technology. I'll say not quite gone awry, but certainly being used in ways that weren't previously intended.
And so just trying to get out in front of some of that becomes really important. And so quite frankly, part of our job is to create a consistent and coordinated approach to governing our AI. And ensuring that it's an all hands on deck approach to how we message around this sort of thing, and how we design into our technology kind of ethical practice, and so on.
So that's kind of the role. As you said we are newly formed. And quite frankly, still challenging the scopes-- the limits of our scope, and understanding what will be in and out, and that sort of thing.
GREG HORN: Fantastic. And so you mentioned that this idea of why you need to look at bias in analytics. I do a lot of presenting on this subject myself, actually. And I put up some examples. One of the things that always gets people thinking first of all, and it kind of helps frame the conversation, is where things have gone wrong in this space in the past.
So can you give me a quick example of something that kind of shows why we need this, because it didn't work somewhere?
REGGIE TOWNSEND: Yeah. And quite frankly, this is the squeaky wheel gets the oil kind of phenomena. There are a lot of infamous examples. I won't name names of companies. Just-- that then won't be useful. But thinking about hiring practices that have gone upside down or discriminatory lending or highly questionable policing.
And you know judgment rendered as it relates to sentencing and those sorts of things. Like there's-- the AI-- and we should probably spend some time, Greg, kind of defining what we mean by that. But you know where it shows up, where these decisions are being made with the use of technology show up in many facets of our lives.
And yeah. The areas where it's gone horribly badly have all gotten a lot of attention and necessarily so. And so, yeah. There are a lot of AI gone wrong stories that we can point to.
GREG HORN: And I like to be optimistic in these things. So tell me a bit about where it's gone right. And I'd like to spend a bit more time on this. Because I think that where it's gone right kind of proves not only the need for your group, but the need for continuous development as well.
REGGIE TOWNSEND: Yeah. So if it's OK, let me spend a little bit of time just-- I don't know who's listening, right. So it's important that we get baseline on AI.
GREG HORN: Absolutely.
REGGIE TOWNSEND: You know, I'm a fan of thinking about AI kind of as a composite. Everything from the ingestion of data. And, quite frankly, you know surveying and all those sorts of things that go into the creation of a quote unquote "data set." All the way through the analytic modeling required, so the descriptive and predictive analytics.
All the way through the visualization of that analytic load and the decisions made as a result of it. And so on. So there's a whole lifecycle, at least the analytic lifecycle. But really the market is defining that more broadly as AI these days. Previously, you know, AI was associated with cyborg robots, right.
People think terminator and that sort of thing. But one could arguably say that your bank ATM is AI, right. We can go there. But it's important to kind of baseline that. Because throughout all of these examples, what's core to each example is this concept of an algorithm. Or basically it's a set of instructions, right.
And we're programming computers to make decisions on the basis of some input, right. That's the fundamentals. And so while there are a number of examples as we've already cited, where these instructions have been either propagated with data that was flawed in some way, shape, or form.
Or decisions were rendered in ways that may not have been completely thought through before they were established, right. Before those decisions were made in such a way that they might potentially hurt people, right. Those decisions, obviously as I said, are horrible. But at the same time there are decisions sets that I use every single day, that go well.
So you know, like I say, you know your bank ATM. If you've ever flown on an airplane chances are you've had a route that's been optimized for that flight, right. There's decision science or data science or AI built into that. If you've ever-- Heck. One of my other hobbies is I like to DJ, right. And I love music.
And so during the pandemic where we're shut down watching videos of different DJs and getting recommendations on new people that I might want to hear, right. That's AI or recommendation engines. If anyone has ever been evacuated, because of inclement weather coming. Tornadoes, hurricanes, et cetera. Those are forecast models that are used, that's AI at work.
And so I think the takeaway, and then I'll pause here Greg, is when it works we don't say, yay, AI, right? Right. We just say, hey, the plane went up and it came down. And it was really uneventful. And that's a good thing, right. But when it goes wrong, than we hear a lot about it. And again, necessarily so. So it's important to kind of increase our levels of literacy around what it is, where it shows up, and those sorts of things. In addition, to being able to know how to optimize it, manipulate it, et cetera, et cetera.
GREG HORN: Yeah. It's interesting. And I think it's important to know that in healthcare, life sciences, we can learn a lot from other industries as well. I-- every time I go to Las Vegas I end up in a dreadful hotel room. And that is AI in action. They know that I'm not a big gambler. They know I'm unlikely to kind of do much for the profits of Vegas.
So they put me in a room that faces the dumpsters. And if they get that decision wrong it doesn't necessarily particularly matter. But in health care there are much more consequences to getting it wrong. So is that the kind of thing you're looking at in your practice? Talk a little bit about some of the methodology you're going to use. And how are you going to measure success?
How do if you're actually doing the right thing with this?
REGGIE TOWNSEND: So this is a good question. Some of this is still being defined, to be quite honest with you. But one of the best practices that we have adhered to, just initially the establishment of a set of principles. So if you think about it from this vantage point, principles drive your values, values drives your behaviors.
And so from a principles perspective we are starting everything with a human-centric point of view, right. So what is it that is going to promote well-being, and human agency, and equity, right. So my challenge is always to the team is say, we should always start with the most vulnerable populations in mind.
Because if you take care of the most vulnerable, than everyone else is generally going to be OK. So if we can, in our decisions-- and I'm talking about from the point of design, through development, deployment, et cetera. If we always take a pause and consider the most vulnerable impacted by the technology, the decision, the process, et cetera, then we're starting in the right place.
And then dotted around that human-centricity is topics like transparency, and privacy, and security, and accountability, inclusivity, robustness. And so starting with those principles in mind, Greg, we then start to look at how those principles, or the attributes associated with those principles, show up in our technology.
So one of the things we're doing now is we're actively going through our entire product portfolio. To say, how does it reflect inclusivity, how does it reflect transparency, et cetera, et cetera. And weaving that whole data ethics story throughout the entirety of the portfolio.
GREG HORN: And actually, one other thing just comes to mind is we've been working with data for good for a couple of years now, actually. Is that something you're going to be now having an influence over as well, where we look at those projects? I think we've done some great work. Particularly, stuff I've been involved within the mental health space has been both interesting, but also caused a lot of change as well.
So would you work with that team too?
REGGIE TOWNSEND: Yeah. In some respects. It's important to see this as kind of two sides, of perhaps, one coin. Data for good is typically associated with corporate social responsibility. One might argue it's part of a philanthropic attitude, right. And that's all good. That's core to who we are as a company. It's absolutely necessary.
What we're doing with the data ethics practice is baking this idea of ethics into our commercial interest. So that's a principally distinct set of activities. So on one hand, you put in a volunteer effort behind trying to do the right thing. On the other hand, you're doing the right thing, because it's also in your economic best interest.
And so two sides of the same coin is how I see those two activities.
GREG HORN: That's really interesting to hear. I think it's a very important distinction, as well. So I'm really glad we kind of touched in on that. And then, sort of building on that actually then, so what excites you most about the future of the data and ethics practice within SAS?
REGGIE TOWNSEND: You know, so I tend to be a relatively hopeful guy.
[LAUGHTER]
REGGIE TOWNSEND: I think that what-- I want to use the broader we. This is not just about our practice, this statement. This is the broader we. If we get this right, and this being kind of the proper development and deployment of AI related technologies, then we really have the opportunity to make a tangible difference in the lives of people.
And it's important to know that, from an ethics perspective, if we get it right and Microsoft gets it wrong then everybody loses. If Google gets it right and we get it wrong, everybody-- like this is a community, man, of people who need to be contributing to a set of standards and expectations around what ethics means and how ethics shows up in our lives.
Both the digital lives, our physical lives, et cetera. So you know I'm excited that there is a community of folks now who are participating in this establishment of, not just of principles-- every company has-- a lot of companies anyway, are developing their own principles similar to what we have. We're all to some extent offering recommendations to our legislators, et cetera.
So I think there is a groundswell of activity around this. And I think we'll get it right. You only have to look over the course of human history to see how new trends introduce themselves. And you go through this curation process. Almost how you learn about in team dynamics. The storming, forming, norming. Like, we're in the process of storming right now as an industry.
And so I feel really confident that we'll form a norm, over time. Where I get really interested slash concern is, is this idea of forming and norming around objectivity, sufficient transparency, the lack of bias, right. Because the last thing we want to do is fortify the systems of old, into the new digital systems of the future, right.
We've learned, well we should have learned, enough lessons about what has not gone well about our past. To try to mitigate against that, such that we can build on a brighter possibility for every single human being.
GREG HORN: That's really interesting. That makes me think a bit about, a couple of things you mentioned, made me think about the episode we did with Dr. Winn, from the Massey Cancer Center and that trust. And he talked about something-- you've had a chance to hear that. What are your reflections on what he talked about in that and how does it relate to your work?
REGGIE TOWNSEND: Yeah. So one of the big takeaways for me, in listening to Dr. Winn piece, there's his idea around, I'll call it leveling out the medical information, the knowledge base, and filtering out some of the human bias that lends itself to that medical cannon.
You know he talked about this idea of, and correct me I forget the term that he used, but this idea of race being associated with certain diseases, right. You know and I really identify with that, because you know growing up in Chicago-- I didn't know it at the time, but I grew up in a food desert, right.
It was not uncommon to hear like my grandmother to say, you know we're going to go out to this neighboring town. You know where the quote unquote "white folk" live. Because there were better groceries, right. They had better produce. When you drove around where we were from, grocery stores were relatively rare.
And it seemed that the produce was questionable and some of the stuff that you get from the butcher was not necessarily the best. And you drive up and down the streets. And you see a lot of places to go and buy fried foods, and liquor, and these sorts of things. Didn't have fine dining establishments. And if you did, they were extremely rare. And didn't last, right. Didn't stay in business for long.
And so we have to start asking ourselves some hard questions and say, do people who are economically deprived just really like fried food? [LAUGHTER] Is that the issue? [LAUGHTER] It seems really, kind of, awkward. Or is there something in the design? And I think enough scholars have proven that there's something in the design. And so I think where his point really starts to become illustrative is, well if you are subject to-- because, by the way, if you have little to no transportation and you're subject to eating these foods that are in your immediate surroundings, medical science has taught us that just enough fat your cholesterol levels will go up.
And so is it that economically deprived people, or African-American people as an example, are more conditioned for hypertension or more conditioned for diabetes? No. [LAUGHTER] It's just their food supply is messed up, right? And if you give them better food, guess what, the levels of hypertension and diabetes go down. So just starting off with data sets that are informed with this idea that there's some presupposition starts off wrong, right.
And so when you start programming AI systems with that kind of data, guess what you're going to get? You're going to get decisions based on that bias. And so part of our job is to make sure that we're properly informed with fact. That we're properly informed and can provide historical context.
And, as best we can, try to inform the technology to look for those things and to weed out fact from fiction.
GREG HORN: Yeah. That's really interesting. I think Dr. Winn referred to the idea of space versus race in his piece. I think you've just really eloquently summed that up. That was great. Thank you, Reggie. Just one more question before we wrap up today. I always like to think about the future. And think about what's going to happen next.
So when you talk about ethical AI, how is it going to develop in the next six months, year, however long. How are we going to get better social and environmental justice on the back of better AI?
REGGIE TOWNSEND: Wow, that's a big question. I-- so one, I know that ethical AI, responsible AI, those are kind of industry accepted terms. But I like to flip it on its head and say, what's the alternative, unethical or irresponsible? Like, let's just call it AI. And just like we call it cars. We don't say ethical cars or we don't say responsible electricity, we just call it cars. [LAUGHTER]
Now certainly, we put seat belts in cars. We put sheathing over wiring, just because that's the responsible thing to do to keep from hurting people. And hopefully we will get to that point with this AI conversation. And I only point that out to say, we might be doing ourselves a disservice with the semantics here.
So I just kind of say that out loud for any evangelists out there working on AI. But to answer your question, it will lead to a more objective-- if done well, a more objective future. It's important to note that we're having a conversation right now largely about technology. Technology, unto itself, will not be the cure.
These are human issues that we're dealing with. And so it's going to take human beings to show up and be better and want to be better. In addition, to technology that augments us and helps us in our decision making. In addition, to putting processes around some of our decision making. The kind of checks and balances in corporate spaces or laws in governmental spaces, et cetera.
So you know there's a lot of work to be done around the technology, in addition to the technology. But I'm hopeful. Like I said earlier, I'm a hopeful dude. So if we show up as technologists with something that allows us to create a more objective, a more equitable future, then I'm hopeful that the rest of the human beings around us will jump on board.
And try to make the world-- this sounds really trite. Make the world a better place, right. [LAUGHTER] But that's real, right. I think there are too many people whose potential goes untapped, who don't live lives that are thriving lives. And maybe we can just be a small part of making that better for them.
GREG HORN: Brilliant. Thank you. Thank you, Reggie. That's really inspiring. It's great to hear at the end there. And thanks for all your insights and discussions on the episode today. So now it's over to you, our audience. We welcome your questions and comments at thehealthpostpodcast@sas.com.
Also, don't forget you can comment on the YouTube channel as well. Let's particularly think about this idea that, yeah, we don't talk about safe cars anymore. Like, how do we make our AI just AI and ensure that everything we do is best practice. I'd be really interested to get comments and questions along those lines, as well.
So please remember to subscribe through your usual podcast channel or through the YouTube channel, so that you make sure you get future episodes. And we look forward to seeing you back in the future. As a reminder, my name's been Greg Horn. I've been your host today. Thank you, very much, for joining myself and Reggie. And we'll welcome to you to another episode, very soon. Thank you, bye.
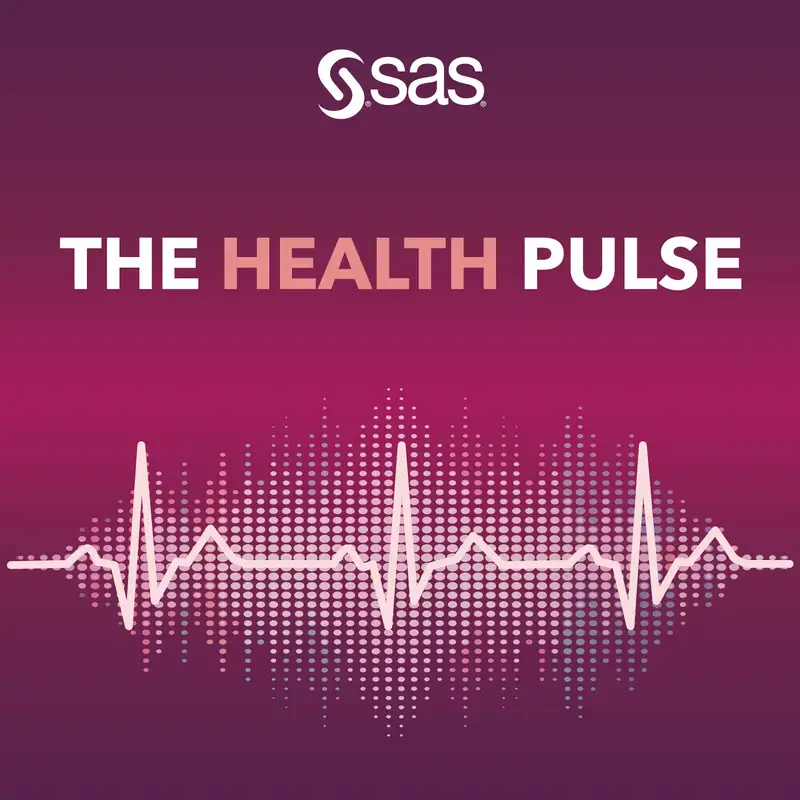