The Health Pulse S3E12: From radical to routine: The use of Bayesian statistics in clinical trials
ALEX MUSBURGER: Have you ever felt like you were 10 years ahead in your business ventures? Today we get to speak to a leader who made a bet years ago on technology that would help predict the future success of clinical trials, and his company was recently acquired proving him right.
I'm your host, Alex Musburger in season three of the Health Pulse podcast and YouTube series, we get to celebrate leaders making a difference in health care and life sciences. Today we get to celebrate Bruno Boulanger, global head of statistics and data science at PharmaLex. Welcome, Bruno.
BRUNO BOULANGER: Welcome, Alex. Thank you, pleasure to be here.
ALEX MUSBURGER: So let's talk about this bet that you made. You started a company, Arlanda years ago that was recently acquired, or acquired several years ago by PharmaLex. Can you tell us a little bit about that journey of how you came about starting it? Maybe you were always an entrepreneur, or was this your first venture?
BRUNO BOULANGER: Well, it was not my first venture to be honest. But this one was probably the core of the discussion we're having today and probably a very interesting one. Indeed, I spent close to 20 years, 18 years exactly in the pharmaceutical industry after a few years at university, working in different areas during my time at big pharma, and particularly in what we call early clinical phase, meaning transitioning from preclinical to phase one, phase two, and to use different type of techniques of modeling that we call translational science.
But at that time I was very fascinated by the use and implementing Bayesian statistics to achieve this goal. And finally, I decided, back in 2010 to say, well, why don't we make a consulting company proposing Bayesian statistics primarily to help translational science evaluating, simulating clinical trials and so on.
So that was my idea. And at that time I consulted a lot of friends and colleagues in the field, and make mention about this idea, and all of them told me that it was probably a very unreasonable idea to do that because nobody, or very few people will ever use Bayesian statistic in clinical development. So we are back 12 years ago.
But I knew some company was already interested by Bayesian statistics, so based on this feedback I decided to create my own company with the Idea If nobody believed it, I probably will be alone in this market. Maybe I will die alone on this market. That's another story. But that was the bet I made at that time.
And so it happened to be very successful down the road, I mean because to my big surprise, starting from one to my colleagues at the beginning, we rapidly end up in the range of 20-25 statistician after six, seven years.
And we finally got contacted by PharmaLex in 2018 to join the group PharmaLex. So I'm saying maybe to mention is that the fact that we were using and applying Bayesian statistics, it opened unexpected doors in front of us, and the unexpected doors was being involved into what we call the CMNC statistics. I mean the statistics for the development and process of bioercy. Everything is linked to the development of a product, the physical product. And as well, different types of involvement into discovery. And so the reason that the company becomes so large, not only because of clinical, but because we extend to the CMNC and we extend to the discovery.
Today, this group is probably unique, I think, because we work from early discovery statistics down to health economics, but applying Bayesian statistics all the way through.
ALEX MAIERSPERGER: I love how you talked about the unreasonable idea. I think as we look back in history, probably most of the best businesses started with those big bets on unreasonable ideas. So congratulations on your success and on the growth. You talked about starting in that preclinical and early clinical phase in the clinical trial operations space, with a few employees, then dozens of employees. And now, your team, what you mentioned has that range of consulting services, regulatory affairs, pharmacovigilance. Are you just solving bigger problems now than you originally started? Or is it the same work with 10 as it is with now thousands of employees?
BRUNO BOULANGER: Yeah. So PharmaLex was originally a regulatory company. And so now we are part of a larger group, PharmaLex. But within PharmaLex, in statistics and data science, we're a group close to 70 statisticians. OK. The interest of working with different colleagues in different areas is that it allows us to dive into more deeply into several types of problems.
So the diversity of the problem we have to address is becoming broader than before, not only in the clinical setting. Because now, we are addressing a more challenging situation in clinical setting, following what's happening in the pharma industry, like rare disease development. But also, what we can see-- and the reason I mentioned the CMNC side is that there are more and more challenging technologies used by the biopharma industry.
We see a lot of cell therapy, meaning challenge in the cell culture. For example, there is way more technologies that are used in the biopharma industry. And therefore, we need to understand how to integrate those new technologies to make decision. And that's where the patient statistics is coming into the goal. Because you mentioned about Bayesian statistics, it means, also, evaluating probability of success, but also decision-making process.
ALEX MAIERSPERGER: You mentioned rare diseases. And rare disease drug development has certainly been in the headlines. I think it looks like there's a real chance we have to develop precise and targeted drugs, down to this individual has this condition and needs this exact drug that's going to match their unique condition. How do you predict future success in rare diseases and in the discovery and the development of drugs? And is this something where major progress has been made or is about to be made? Can you tell us a little bit more about the rare disease space?
BRUNO BOULANGER: Yeah. Yeah, the rare disease is indeed a very challenging situation where you only have a few patients. Fortunately, there are few patients only having this type of disease, for example. So it means the ability to make a classical clinical development is out of scope. There is no way you can do that. But that's the challenge.
Now, this is where the Bayesian statistic is coming into the picture. Why? Why? Because in Bayesian statistics, they are, I would say, four parts there. The first part is what we call the PIO. And the PIO, it is all the knowledge you have. PIO doing the study That's the idea.
And so what it means, particularly in the rare disease area, if there are only a few patients, the other side of the aspect is that those few patients are, in a way, very well known. And they are existing in the registry. Because a lot of hospitals are interconnected and they exchange a lot of data. About how can we deal with those patients? Meaning we have a lot of natural history information from those patients, in most of the cases which is made available.
So all this historical information from past patients and current patients is available to build the PIO knowledge. This is the way a patient with this disease is going to evolve in the future. That's the PIO information. Now, the Bayesian statistic will allow you to combine and to enrich the study you are going to do with this PIO of information.
For example, you say we have seen that the patients in rare diseases are evolving in such a way, if after the treatment we apply, we see a change in the trajectory of the disease progression, that may be a sign that the treatment is effective in some way.
And therefore, the third part, I will say, for the Bayesian statistic, which is the probability. Which is what's the probability that the new treatment is better than the previous standard of care? OK. That's really specific to the Bayesian statistics, as opposed to the classical statistics. Bayesian statistics try to figure out what's the probability of an improvement, given The data, given the PIO and historical knowledge we have?
And now comes into the game the fourth part. And the fourth part is what we call the predictive distribution. And what the predictive distribution is telling you, given the result you have observed in your clinical trial, given the historical data, what is the probability of future patients not yet included in the clinical trial? What is the probability a future patient may benefit from this treatment, given everything you know before?
And that's something which is becoming extremely critical in the rare disease because you use this predictive distribution to be able to say, yes, given the limited small data information we have, small data, and given the large data, big data we have in natural history, this is how we can predict if future patients may benefit of this new treatment. And that's really something very fundamental.
ALEX MAIERSPERGER: Bruno, there's obviously a lot of challenges in the world. And you mentioned challenges throughout of even parsing the data and getting it. And then there's challenges in global strife, in economic conditions, and in global disease burden on both the health care and life sciences sides. What's something that keeps you optimistic? And what are you optimistic about in the future of health?
BRUNO BOULANGER: Yeah. I'm really optimistic for various reasons. And I think there are two momentums right now that may justify my personal optimism. The first one, I'm working now since 30 years in the pharma industry. And what I do observe for the benefit of the patient is the wider diversity of treatment.
Previously, it was just about small molecules. And then came large molecules, monoclonal antibody, and then vaccine, and then therapeutic vaccine. And now we have cell therapy, gene therapy, and so on. And it's increasing. There are plenty of new ideas coming on board. Meaning there is an increase of the diversity of the means we can treat the patient.
And I think it's very promising because we can see from small molecules to monoclonal antibodies, the evolution that it happens, and then from vaccine to therapeutic vaccine as well. And each time there is new thinking, and new technology, and new approaches coming on board. There is always unmet needs that are totally fulfilled. So that's really promising because the way to tackle different type of debilitating diseases is really improving. So I'm optimistic on this side.
The challenge-- and also I'm optimistic, also, on the other side-- is that with all those new means, new challenges, new problems that we need to solve. But in the same time, there is this numerical revolution which is happening in the pharma, just like in any other industry. Meaning we have the ability here to collect plenty of data to understand what's happening to very rich data about many aspects.
ALEX MAIERSPERGER: Certainly exciting times. And, Bruno, thank you so much for spending a little bit of time with us today. And thank you for the big bet that you placed in Arlanda, and for the success that you've enjoyed, and for the benefit to us all as drugs are discovered and brought to market.
[MUSIC PLAYING]
So I appreciate you.
BRUNO BOULANGER: Yeah, thank you very much for the invitation and taking me for the very nice discussion, yep.
ALEX MAIERSPERGER: And to all the listeners and viewers, we know that there's so much going on and there's infinite demands on your time. We appreciate you being here. And we invite you to the comments, either down here on YouTube or at our email address, thehealthpulsepodcast@sas.com.
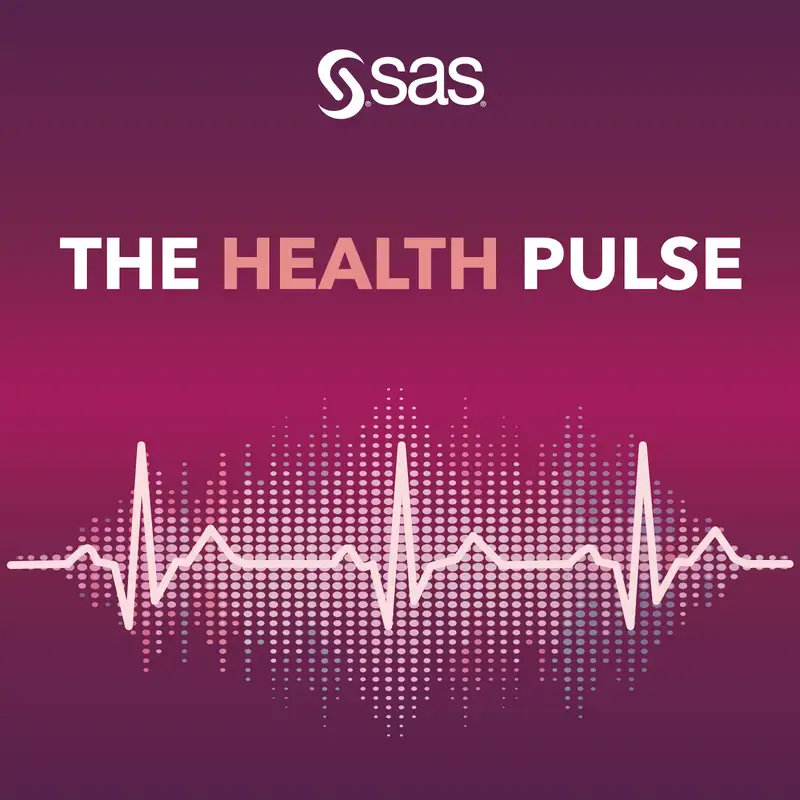