Balancing data, analysis and speed in business decision making
ALEX MAIERSPERGER: What does the rise of remote work, writing books, and applying AI have in common? Today we're going to find out. I'm your host, Alex Maiersperger. And today on the SAS Health Pulse Podcast we welcome Steven Lehmann, head of data science and analytics strategy for EMEA at Johnson & Johnson and author of Digital Jackpot, How to Win the Digital Gamble. Welcome, Steven.
STEVEN LEHMANN: Hi, Alex. Thanks for having me.
ALEX MAIERSPERGER: So you're a data-science leader. Have you done the data science to find out how many pharma leaders have written books? It's got to be a small group, right? So what drove you to become an author?
STEVEN LEHMANN: I mean, obviously, it's a small group. And if you see in terms of how much royalties Amazon takes away from you, it's going to be an even smaller number. And it's definitely not something that you do to get rich.
But it was a quite interesting time because I directly wrote the book of the beginning of COVID. And you might know the old saying of, never waste a good crisis. It was exactly that. Two hours of not being stuck in traffic each day. How about I use the time productively? And I thought about, what can I do? Let's write a book.
Let's interview a lot of leaders in data science and digital and figure out what actually works because it was always fascinating. I've always been going to conferences, talking to people. And if you hear people talk, it all sounds amazing. Every project is a success. Everything you see, everyone is so advanced. And I always felt bad.
And then afterwards, you have the after-show dinner parties and drinks. And latest after the second bottle of wine, everyone went like, actually, it's so crap, or we are not getting anywhere, or we are not getting far, or it works as a proof of concept, but no one has adopted it. And the number of real cases that were successful were actually small.
But once they hit, they were massive changes in the way of thinking, working, and everything around. So I wrote the book of Digital Jackpot to see, is digital actually a gamble? How do I win the gamble? And I did the analogy on poker of how you play with your hand. Sometimes you have good cards, bad cards. You might have good data, bad data.
There are different ways of playing different techniques. And you have a lot of players in the industry that are just bluffing their way into how amazing everything is. And you see the same with the amount of Chat GPT startups that are going all around in the world. And it's quite interesting, but sad at the same time sometimes.
ALEX MAIERSPERGER: One of the things I've joked about is that we're in a headline era. And so nobody reads the sort of details of the article. And it sounds like you dove deep into those details. So the headlines, what you said is, everything sounds sort of perfect. And everyone's doing better than we are, better than I am.
So you've interviewed thousands of leaders across different organizations about how they're using advanced analytics and AI to drive decisions. One of the things you wrote in your book, one of the lessons you shared, is that the goal is not to be 100% accurate. The goal is to be less wrong. It seems like you're in an industry that's held to that 100% accurate ideal. Can you explain what you mean by less wrong?
STEVEN LEHMANN: Yes. And there's so many different levels in business. And what unfortunately happens is, we do everything one way or the other. The idea of the goal is not to be right, but to be less wrong, is especially more on the business side. So in a clinical R&D study there's no way to not be as right as you can get.
But in the business angle, it's a different world. In business, being right but too late is as bad as being wrong at the wrong time. So there are so many ideas. And I have been in the industry for a long time. I've seen a lot of projects successes and failures, depending on whom you ask within my own companies, but also in the competition.
And the ranges are quite wide. You might have people who say, I have today a forecast that is 70% accurate that we do manually. The AI model is 95% accurate. But we complain it's not good enough because it's 5% missing.
Or you have other cases-- and we use a principle that is called the 70-40 model, where we say, if you have less than 40% of information, don't take a decision because you will be wrong. But if you have more than 70% of information, in business you're usually late.
You've seen that during the beginning of COVID. No one knew where COVID is evolving to it. It could have been a six-week short topic. Or it could have been the two-year exercise that we have seen. And it was quite intense on people.
But the companies that took the best decisions at the beginning to react with the information they had, they came out much, much stronger. You see that on company level. And within a company, you see that on country level or in department level.
Those that took with the maximum of data that they could have at the moment, a decision always came out right. Because in the end, it's a bit like the example of poker. Some hands you win. Some hands you lose. Not playing the hands always means you lose at the end. Because over time other people win, where you could have taken the right decisions.
And you see that all across. You see that in management reporting, where you can discuss numbers till the end. And data science is an exciting area. But you can be falling in love with your numbers. You can look for another number and another area and another discussion.
You're almost looking for data for the sake of data. You have topics on, we call them, watermelon KPIs, where people try to have green colors, so outside green, inside red, where you try to make it in a way to make your story fit and try to go further. There's more data. There's another PowerPoint slide, rather than focusing on the actual decisions.
ALEX MAIERSPERGER: Talk about that data for the sake of data. And I imagine that's only gotten worse. Recently, we're in a world where obviously the types and the amount of data we're receiving has greatly exploded. How do you settle that tension between the data-science side and maybe the pure business side?
Data scientists, like you said, are known to want more data. And so how do we get more and more? And business users want to make good decisions. But they also maybe want to make faster decisions.
STEVEN LEHMANN: I can tell you a couple of examples here. The first one is, the key principle or technique we use is decision elasticity. Decision elasticity is calculating how much of your data could be wrong, and you would still take the same decision. Because what you are interested in is--
Quite often in the business discussion you make a recommendation. We need to increase the headcount on the field force. We need to invest more in that channel. We need to have a new model for x, y, and z.
And then the question is, do people trust your data? And the belief is, I want more data. And more data makes it more accurate. But you've never actually seen that. So what we do with decision elasticity is to say, hey, the recommendation is increase this investment by 20%. And this recommendation still stands even if 18%, 20%, or 30% of your data would be random, wrong, or whatever.
Because you love as a data scientist, everyone complains the data is not good. The quality is not there. People have not maintained it. We don't have the right culture. But in a kind of business context, you're not working in a perfect environment and live in a bubble where everything is perfect. You have to adapt to how good is good enough.
And many of the data scientists have to develop in that area once they go into business. If you come in academia world or research world, they are, I would say, their approach of more data has definitely devalued. In a business context, the only thing that matters is the impact that you can create with the recommendations that you do.
It truly does not matter what the underlying ideas are or how much more data-- and can I get 1% more accurate if I just buy half a million more of data points? Or if we all start cleaning our data? If the outcome is the exact same. And we gave that as an example in a topic like a thermometer, where you see what's the temperatures outside.
A thermometer that lies in the sun and the thermometer that lies in the shadow will show different numbers. It does not mean that the KPI of thermometer is wrong or that you need to have 17 additional thermometers around your house if the question is, do I need a pullover? It also means if your problem is, do I need a pullover? Thermometer is a great answer or a great KPI to help you.
But if your problem is actually, do I need a rain jacket? A thermometer is quite useless. And companies have this tendency to discuss the data for the sake of data. And you start by discussing the data, rather than whether it is relevant for your underlying question because a KPI is useless, unless it has the context that you want to generate.
And data science really goes three, four steps further. And we have a much higher accountability in data science to go towards action, to go towards impact. You see it in the industry, as data science teams in pharma will get the sales target, or at least the sales impact target. You see that they become accountable not just for the analysis itself, but the actual decision that comes out of it. You see that a model can be statistically right and business-wise completely useless.
We had a small group of students who did an amazing work on forecasting. And they forecasted a trillion loss in sales, which obviously you will never present to your CFO. But in this case, the r-value and p-value look good. Now, that does not mean you can actually put that into a business context. And sometimes an easy regression can solve your problem the same way as a truly advanced deep-learning model.
But the balance between this, the balance between how do I ensure what we do in data science creates impact is one of the biggest topics the industry has to face, not just the industry or pharma, but every industry. But especially pharma needs to take it a step further. And you have to see pharma-- a lot of the data science comes out of pharma, out of clinical.
A/B testing that we do in marketing now is long there in clinical trials. Randomized trials, obviously, we do in marketing to test campaigns. The industry has done a lot of things. But now we take it to the next level of actually creating the right level of impact. And data science matures out of the curve, where it was interesting, sexy, nice to, where can I actually create a real impact?
In the past, we would have called data science statistics. And in a business context no one would have joined a meeting that was about statistics. Now if you have a meeting about data science, the room is full and booked in the first 15 minutes the moment you offer it. If you offer a statistic course, no one in management comes. But data science goes to a whole new level if you employ it right and focus on the stories the decisions at a whole different level.
ALEX MAIERSPERGER: I heard you use the word students. And then you talked about storytelling and tying the data science and the data scientist to the overall end impact. Is that an organizational skill set? Is that something you teach your team? Is that something that's part of now the data science education curriculum? Is it something we need to be training data scientists to be able to do, to be able to tell that story of, how much data is enough data?
STEVEN LEHMANN: You have currently two sorts of schools in this. One is, I hire a special profile. They're usually called a translator who actually are between a data scientist and the business. And you have those that believe data scientists need to upskill to the level to tell the right stories with the data.
Because in the end if you have the right answer, someone does not take the right decision because you have not told the right story. That's one of the most depressing things in your life. There's nothing worse than saying, I was right, but no one listened to me because I didn't tell the story right.
I knew COVID is going to take that amount of time. But no one believed how I told the story. In business, it's about decision-making. If you can't influence the decision, it's a key problem. And you have that along the whole value chain.
You have to think at topics like forecasting. It's an organizational capability for us to reframe questions. Forecasting is an easy example. Today, how do you measure success of forecasting? It's how accurate you were.
Now that also means if you say you are going to underperform 20%, and you're accurately forecasted we are going to be 20 million, 200 million below budget, congratulation for being accurate of telling us we are not making our numbers and not helping us to solve the problem.
We mentioned it as the Titanic. Like, if I accurately can forecast when you hit the iceberg, and I'm happy the moment you hit the iceberg, because that's when I did my job well, that kind of feels wrong.
So data science in some areas, you can see, it's being evolved from data science to-- we have a separate group on decision science that looks at psychology combined with storytelling, combined with the data science on how do you actually influence the decision.
Because what do you want more? To know when you hit the iceberg? Or how to avoid the iceberg? I'm not 100% sure you're going to hit the iceberg. But, well, by the time I'm sure, it's too late.
ALEX MAIERSPERGER: That decision elasticity, the amount of data and the timing, I love that, very insightful of just the timing matters so much and then tying it to impact. The Titanic example is a great one. Right now in the headlines there's a lot of fear and misconception about AI.
And so I think people, at least in the headlines that you read, it seems like AI is going to be the thing that's going to get us either better predictions or better ability to make the end impact. Is the fear justified? Are most organizations already getting the value out of AI that many of us think they are?
STEVEN LEHMANN: Well, here you really need to distinguish two worlds. If you ask most companies, first they would say, yes, we are getting all the value out. Again, if you discuss a bit further, most are not at that level yet. 90% of all the analytics that are out there in the market of truly AI-called models are probably a regression you could have done in Excel.
There are some very good models and very good products that are valuable. And there's nothing wrong with it. But we are very far away in most business contexts that you are afraid about how AI is going to take over. It's a bit like, you're not going to the fitness studio because you're afraid you're going to look like a bodybuilder.
There's a big range between where the fears are justified. And there's the reality where companies are today. And you can take still a long way in that journey to improve things and take better decisions and use AI where it creates high value before you reach the point where we should be afraid.
And Chat GPT is, I think, a good example where we start going in an area where people get the feeling it gets nervous. But if you look how many companies have developed their own version of Chat GPT at that level of quality, the number is-- probably you can count it at one or two hands. And it's probably not the ones who are in a business industry.
Just data science in the end is nothing else than, how do I take better decisions with data with the right approaches? It includes topics like game theory. It includes topics like, how do I use my external data? Most companies have not reached the level where they used external data from Google, from social, from different areas to the point where it creates impact.
Every word document that you write in a company is data that contains knowledge. Most PowerPoints were documents were actually lost. And people will not even know where they were after they've been presented. They are so much hidden knowledge in a company that data science can help to untap.
You have cases where people start looking into how Google can help me. Or how can I combine Google data with the data that we have locally in our companies to make better decisions of, where's the next refugee crisis? Do I need to change my medicine and my marketing models to support the refugee groups in the best way to create the right impact for patients?
You can model out things at a rate that we have never been able to before. And that level of thinking and that level of development is strong. Chat GPT, you can create content. You can write personalized messages. You have Daly which helps you create marketing materials at a faster level. You have insights that you get from these chat bots that you can use at a whole different level if you use it right.
And we mention it also in the context of artificial intelligence, data science, these are great tools. But they're just that. You will not be replaced by AI in the majority of cases. There's very few cases where this will happen any time soon. But you will be replaced by people who used the technology better than you. And we've seen that.
AI is not better than senior forecasters who do forecasting for sales. And you can look at any industry. AI is almost at the same level. But then in the company you come into the fight, who is better? Is manual still 1% better than my automated one?
And then you do one check is, a junior forecaster with AI outperforms both. You have the same interests. You have the same in different parts of the industry. Marketeers who will use Chat GPT better than others will succeed better. Those that take the right insights out of data science will operate faster, better, or smarter in everything that they do.
And that level of thinking is really where you play with data science, with everything in that space, and where decision signs becomes a portfolio management of, some topics you win. Some topics you lose. But you really try to, how as a whole I can manage to succeed for my entire company.
ALEX MAIERSPERGER: I love that take. Very often it's technology versus people. That seems very much a technology and people, or people and technology insight. So really appreciate that.
You've talked a lot about impact. And so just talking about career-wise, you've built facial-recognition software at 14. You've helped global multinational organizations set strategy for emerging technology. You've interviewed organizations all across different industries, finding out what they're doing in AI. You wrote a book. And so right now, what excites you? What's going to contribute the most to a healthier future?
STEVEN LEHMANN: The most that excites me and especially the space is less the technology, but the human evolution linked to the technology, the ability to take decisions on a whole new level that we never had the possibility before. That, I find truly exciting.
The ability of topics like ethics in AI, we talk about it as a risk. I see it more as an opportunity. We can have a whole level of ethical AI and ethical decision-making that is embedded into the system in the way of thinking into the process that we never had before. The ability to find hidden biases that normally we would have never found.
There's a lot of examples where we have seen-- we think we are not biased. But we actually create part of the problem because we don't look behind. And AI helps us to look much more cautiously, how do we make the right choices?
There's a lot in our data that shows how biased we were. But the right choices to do ethically and successfully, it's truly sometimes inspiring. You have cases like, you can build the next best action that recommends a sales rep with whom he needs to talk or she needs to talk. And you have that in all industries.
In the industry of, for example, alcoholic beverage, it's the first time you would include an ethical level that says, we can recommend you to sell it. But if there's an indication that you would increase alcoholism in an area, you have to stop. That's something you would never have done.
Pharma, you have the opioid crisis. The next best action with an ethical model can actually tell you to stop and say, hey, it's not just about, how do I get more sales? Or how do I go? But here's actually something wrong. This is not how the products should be used. This is not how we save patients. This is not how we help patients. And that elevation of it and how people use it, I consider extremely exciting.
ALEX MAIERSPERGER: Steven, we're so fortunate to have had you as a guest here today. We've learned so much. So appreciative of you joining us.
STEVEN LEHMANN: Thank you, Alex. Thank you very much.
ALEX MAIERSPERGER: So you've heard, I think, some tips from poker or tips about poker. You've heard about thermometers, watermelons, even bodybuilding, and the decision elasticity and the ability for data science and data scientists to really tell their stories and be able to impact patient lives and members and consumers.
So stay tuned as we continue to tackle some of health care and life sciences biggest challenges. We'd love to hear the ways that you're driving a healthier future. Please, leave a comment down below or reach out to us, thehealthpulsepodcast.sas.com. We're rooting for you always.
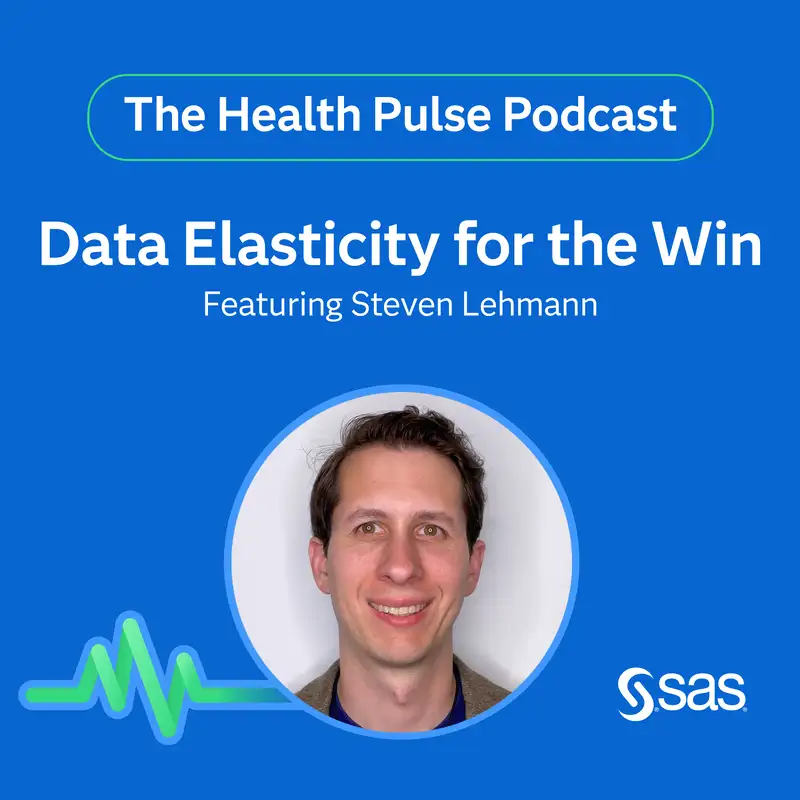