The promise of AI in pharmaceutical manufacturing
ALEX MAIERSPERGER: Heavily-regulated industry and innovation-- can those two things go together? Today, we're going to find out. I'm your host, Alex Maiersperger. And today on the SAS Health Pulse Podcast, we welcome Andy Bayliss, advisory business solutions manager at SAS. Welcome, Andy.
ANDY BAYLISS: Hi, Alex. Great to be here.
ALEX MAIERSPERGER: Andy, we're so fortunate to attract incredible people to SAS. Previous guests from SAS internal-- we've had Olympians, professional athletes, even a movie star. You're a fan favorite here. So what's your background? How did you end up choosing SAS?
ANDY BAYLISS: Thanks, Alex. Well, I've been at SAS for just over 10 years, right? I've got a background in software development. I left university doing a degree in mathematical engineering, which I usually joke with my family means I know very little about maths and even less about engineering.
So I got into the software industry. I spent years working at a car manufacturer, actually, doing data analytics on financial systems there and then moved into software-selling and sales side. And eventually, 10 years ago, I ended up at SAS-- best move I ever made.
I joined because I was drawn by both the technology, the people, and the opportunity from the UK CIO at the time, who sort of said he wanted to build the best team at SAS for supporting the great software that they'd got. And that's really what attracted me.
ALEX MAIERSPERGER: So you have that multi-industry expertise. Looking either across industries or directly on the life science side, is there a standout example where new technology, either software sensors or other aspects, has been applied and is making a difference in the production process?
ANDY BAYLISS: Yeah. I mean, I do a lot of work with the life sciences companies, which is why I'm on the health care podcast, right? But some of the really exciting stuff is happening in health care, manufacturing, and life sciences, right? But the one that always stands out to me is actually with a paper manufacturer, right?
We have a paper manufacturer who takes raw pulp and produces all sorts of different paper products, right? And they've got over-- around-- well, between 10 and 15,000 models in operation monitoring that production process, keeping it on track, making sure the products that they produce are real quality.
I've got some examples from my time at SAS where we're doing it with some manufacturers, right-- life science manufacturers-- but not at that scale. I'd love to get there with the life science manufacturing teams, but we're kind of starting small but really got big aspirations, I think, to take the experiences that I see from SAS in other industries and really bring them into that life sciences manufacturing space.
ALEX MAIERSPERGER: I was recently at an event. And one of the exhibitors was showing a pill with a sensor included in it that was so small-- that you'd take the pill. And it uses that sensor to send a signal either to the manufacturer or directly to the physician confirming that you're taking them. Is that the future, or is that already commonplace now?
ANDY BAYLISS: [LAUGHS] Well, I think we'd all seen those films where everybody gets miniaturized and the submarine gets swallowed, right? I think we're probably a long way away from that. But I think the idea of smart devices-- and the pill that you swallow is one example.
But I think there are lots of smart devices that are out there that are starting to come on the market that actually can generate huge amounts of useful information but only if you can actually analyze it, take it, understand what all those data points are saying, and get some real actionable insight from it.
So it's all very well having millions or billions of data points. In fact, our CIO made a lovely phrase the other day-- in fact, our chief marketing officer. He passed it off to our chief marketing officer for-- one of our new phrases is, how do you take a billion data points and get one single point of usable insight? And I thought that was fantastic because that's exactly what I'm seeing happening in life sciences manufacturing, in manufacturing in general, right-- but taking billions of data points and making useful information out of it.
ALEX MAIERSPERGER: Really interesting because there's-- manufacturing is such a heavily regulated industry, especially in the production of new drugs. And so when you think about the explosion of all of that data and then getting to that one insight-- so you have that heavy regulation of sort of that prescriptive, here's the things that you have to do. But at the same time, there's a lot of opportunity for a healthier future that relies on getting drugs out faster, or better, or cheaper. How do you see that heavy regulation side and innovation going together?
ANDY BAYLISS: Well, I think they have to go together, right? You literally can't do things in the life science industry without making sure it meets the checks and balances that are in place and that we're all so useful-- so used to, right? You get your drugs to medicine-- to market. You're reliant on them having been checked and doing all the statistical analysis to prove they're safe and effective, right? And as that goes through the manufacturing phase, we have to maintain that same level of rigor. Right?
It's really important that you know the checks that have been done, whether they're with simple business logic or more advanced AI machine learning rules-- have been done in a regulated, controlled-- and I suppose, for SAS, one of our things is a trustworthy way, right? We make a huge thing these days about trustworthy analytics. And that applies whether you're dealing with personal data, looking at fraud detection or granting of loans, or whether you're looking at proving that a product is safe and effective or safe and secure for a marketplace.
ALEX MAIERSPERGER: Andy, we had a previous guest talk about proof of concepts. They said their organization will never run another proof of concept because they always work. And so you have that sort of incubation place, where you're willing that proof of concept to work. And then you can often struggle to get it to implement at scale.
And so whether it scales can be successful or not, but that proof of concept always works because that's sort of baked into the process of, we have this smaller team, we have this environment where we're going to make that proof of concept work. And it's not really indicative of if it's going to actually work at scale.
So you've had experience in both-- on the proof of concept side and in actual implementation at scale into the billions of data points. Is that guest's take the right one? Should organizations be taking more decisive action to scale what's working and not worrying about that proof of concept side?
ANDY BAYLISS: So that's interesting. I'm thinking I'd love to work at a place where every proof of concept works, right? So it's definitely not my experience, right? Proof of concepts are there for a purpose, right? But I think what you have to remember is it's keeping the end in mind, right? If you've got something that's got to work at large scale, then that needs to be part of your process.
Typically with the processes that we take-- we engage in a proof of concept, right? We're looking for failing fast and scaling very, very quickly, right? And the beauty of SAS is we're very, very good at scaling things, right-- so the ability to go from a few hundred data points to billions of data points. We know that the software will deliver the results that's needed.
So I think we're fortunate in that, while maybe not every one of my PoCs that I've been personally involved in has been 100% successful, those that we have had success have scaled very, very well. So I do think it is about remembering that if you've got to deploy these things, then they're going to have to run at large volumes. We're not in the industry, really, where you can make lots of experiments just for experiment's sake. They have to have an end in mind. And especially in manufacturing, you need to be repetitive, reliable, and able to run at scale.
ALEX MAIERSPERGER: We've talked a little bit about sensors and cameras, internet of things-- sort of all the new technology that's applied to the manufacturing process. And then you also mentioned that there's organizations who feel some of that pressure, maybe, of data overload-- of, I have to get actual insights from this. Can you talk about that balance of applying technology, getting more data, but maybe not getting to the insight you need? Do you see real examples where all of these sensors and all of these things have caught new things that you didn't know about the process before?
ANDY BAYLISS: Yeah, I think not necessarily, right? I think the thing is-- and certainly in a life science manufacturing space, right? People know their processes. They've been refining these processes. They are experts in manufacturing, right, and in producing extremely reliable processes, right?
So what the sensors allow you to do and the capturing the data at scale allow you to do is to spot issues perhaps before they become really significant or to spot a trend or a deviation that could have an impact further down the line, right? The manufacturing organizations that I work with absolutely know how to manufacture the things they're manufacturing at quality, right? But it's those small deviations that, if they're missed, can have significant impact. Those are the things that machine learning, AI, monitoring, continuous monitoring, and the sensors can help you capture.
And it's just too much information to process, right? If you've got devices going past on a production line at 1,000 a minute a camera can take a picture of everyone, right? But an operator can't look at all those pictures. But the software that we have-- AI machine learning, computer vision software-- can check everyone instantly and then flag up those ones that need looking at by an expert human, right? We have instances like that in manufacturing, in health care. Really, there are so many now where we're using kind of the tools to give extra insight to the human, not replacing the human.
ALEX MAIERSPERGER: We see a lot of challenges in the headlines-- drug shortages, ingredient shortages, different challenges that we see all across the life science industry. What are you optimistic about? What keeps you optimistic for the future?
ANDY BAYLISS: That's a very good question. I think it's the ability to apply technology, I suppose, to solve real problems, to address some of those issues before they become too much of an issue, to provide the insight where there isn't any to start off with. It's about empowering the individual, right?
It's not-- we hear a lot about the fourth Industrial Revolution, don't we, right? And everybody worries and thinks, oh, what's going to happen, right? But we're all much better off having gone through the first three, right? I'm so glad that I'm not working down a mine, for instance, or in a field, right? We've all got the benefits.
And I think what keeps me optimistic is the technology that is evolving so fast, right? And AI is all over the news. Even my friends who don't work in the software industry now want to talk about AI and how it's going to change the world, right?
So what keeps me optimistic, I think, is the fact that we have some amazing technology. We can do some amazing things with it. Not just in the life science industry, although that's where I'm particularly passionate about, I think-- but just the ability to bring new tools, new techniques to different problems to help make everyone's life easier.
And we're in the fortunate position, again, in the life sciences industry that there is a patient at the end of every journey, right? That whether it's my wife who's on some new treatment-- it's all amazing that there's a patient at the end of what we do.
ALEX MAIERSPERGER: Thank you so much, Andy. So I share your optimism for the future and love how you've described how technology has been applied to really make an impact in individuals' lives. That's what you've heard here, is, even with billions of data points, getting to that one insight is so important. And we've heard real examples of how those insights are changing patient lives on a daily basis.
[MUSIC PLAYING]
So stay tuned as we continue to tackle some of health care and life sciences biggest challenges together. We'd love to hear the ways that you are driving a healthier future. Leave a comment on YouTube here below or reach out to us, thehealthpulsepodcast@sas.com. We're rooting for you always.
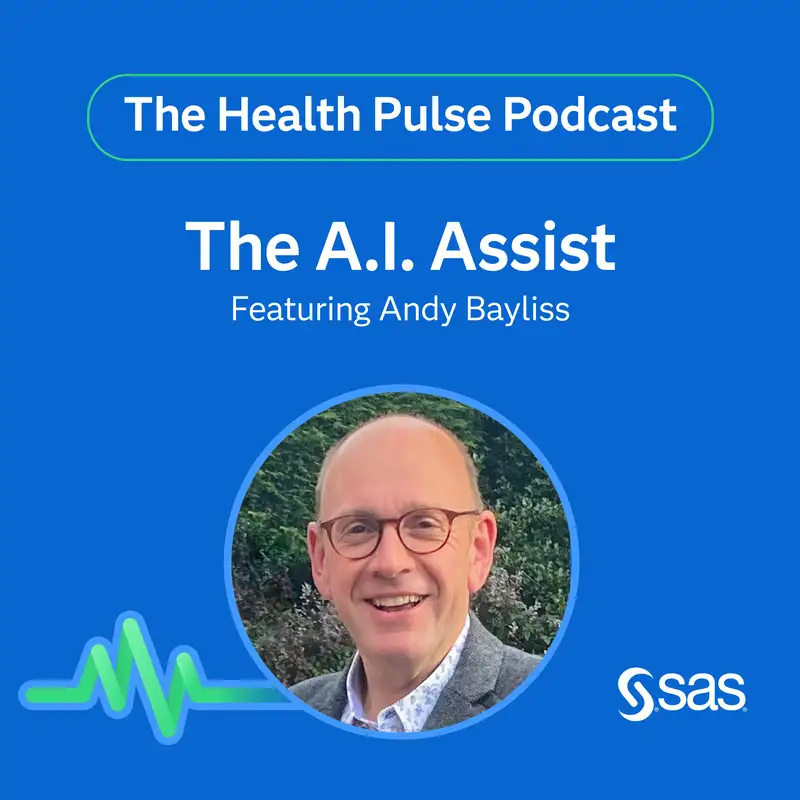