AI, Imaging and the Race to Develop Therapies
ALEX MAIERSPERGER: AI models can already see things in radiology images that the human eye can't. With this, what information could we unlock from scans that have already been performed in the past? Today's guest tells us what this might mean for the future.
[MUSIC PLAYING]
I'm your host, Alex Maiersperger, and today on the Health Pulse Podcast, we welcome Dr. Greg Goldmacher, Associate Vice President, Clinical Research and Head of Clinical Imaging and Pathology at Merck. Welcome, Greg.
GREG GOLDMACHER: Glad to be here. Thanks, Alex.
ALEX MAIERSPERGER: So it's often hard to read through the hype, and right now, we're bombarded with so many of the challenges in the world and in medicine. So we need some good news first. Where is AI making a difference right now?
GREG GOLDMACHER: So it's a great question. And, of course, there is a lot of hype. Radiology has been a really target-rich environment for AI research for a long time because it's already pixels in arrays, data in arrays. And data in arrays is a really easy place to go looking for patterns. And fundamentally what AI is is pattern recognition.
So if you have a nice standardized type of data that you can fit into a pattern-recognizing tool-- and in radiology, we are very fortunate that we have this thing called DICOM, which is a data standard that everybody uses and can be used as a common input, there's a lot of opportunity for developing technology.
And so when I talk to folks about AI, my standard joke is that we were doing this research before ChatGPT made it cool, before that's what everybody was talking about because of LLMs.
So where AI is already making a difference now, a lot of it is in helping-- in radiology, it's mostly in optimizing workflow for radiology, which is basically prioritizing cases and helping organize cases for doctors to read. And the other is in making diagnoses. So for example, screening mammography.
So when I was a radiology resident screening-- there were the very, very beginnings of CAD, Computer-Aided Detection, systems for mammography, but frankly, they were terrible. They would-- anything that they highlighted, it's kind of a coin flip as to whether it meant anything or not.
But AI has made tremendous progress, and computer vision has made tremendous progress. And at this point, the use for diagnostics, such as in looking for tumors in a mammogram, is actually a very good use case. And there's over 100 approved-- FDA-approved products for AI in radiology, and most of them are essentially diagnostic cases.
Now from my point of view sitting in clinical development in pharma, the application of using AI to improve drug development, there's a couple of areas of application for this. So first of all, I mean, AI is being used a ton in early development-- protein folding and drug and target discovery, target validation, candidate screening, that kind of stuff, but that's not where I'm focused.
So in the area, in radiology, so the way you basically could apply AI-- so industrialized pattern recognition for images, is, number one, there's a potential for AI models to see things in scans that human eyes simply can't. And the other is to automate tasks that are labor intensive, and thus, enable some kinds of analyzes to be done at scale in ways that, right now, they could be done, but they're difficult to do economically.
An example of the first type of task-- that is, recognizing things that aren't that are hard for human eyes to see, is the notion of radiomics. So the idea is-- so "-omics" basically refers to rather than looking at one thing, looking at a whole panel. So when you talk about genomics, you're looking at the expression not of one gene, but of, let's say, all the genes.
So similarly in radiomics-- and some people don't like that term because everybody has a genome, but it's a little harder to define what is a radiom, but basically the idea is you've got to scan-- let's say it's a CT scan or an MRI scan, and let's say there's a tumor on the scan. So the way that assessments of whether drugs are working and what's going on with the tumor are done right now it's mostly you just look at how big the tumor is.
But there's a lot of information that's in the scan that is hard to extract visually. It's like textural things, or quantifying the geometry of the margin between the tumor and the normal tissue. How sharp is it? How fuzzy is it? How spiculated is it? And things like that.
So there are features-- so-called features-- basically pixel patterns-- that can be correlated from-- the pattern of pixels on the screen can be correlated with some biological phenomenon, some measure of, let's say in cancer, in the tumor microenvironment. So for example, a measure of inflammation. There's been literature showing that there are pixel patterns that correlate with the degree of inflammation of a tumor.
And that's, of course, really useful because that means that if you were-- you're considering a patient for treatment with some kind of immune therapy, an inflamed tumor is more likely to respond than a non-inflamed tumor. And so that's something where if you have essentially a digital biomarker-- that is, a pattern of pixels that's extracted and that a model can see that the human eye can't see, but that tells you what's going on in the tumor. So that's one application.
The automation of tasks is also a big deal because there are some analyzes-- so for example, there's a growing interest right now in a method, again, in oncology for something called tumor growth kinetic analysis, where instead of just measuring the size of tumors and saying, did it grow by a certain percentage or shrink by a certain percentage and declaring a drug to be working or failing based on that, that's pretty far from the biology.
So the problem is that right now, the decisions that are made on that basis, they have a decent correlation with what you really care about, which is does the drug prolong survival at a large number of patients? But with small numbers of patients, it's actually-- the correlation is not good. And there's a long history in cancer drug development of drugs that look great based on initially making tumors shrink in early-stage trials, and then you get to later trials and they don't improve survival.
So there is another approach that's being pioneered based on tumor growth kinetic analysis, which involves doing a bit more math, measuring more tumors, and ideally measuring the tumors not just with a diameter, but like in terms of the whole volume, which means drawing a boundary in 3D around the tumor. That is certainly possible to do for humans, but it's expensive because radiologists' time is expensive.
Whereas the AI tools that have been developed based on showing AI models examples of tumors that have been drawn by-- with an outline drawn by radiologists and just teaching the computer to draw tumor boundaries on its own.
Now all of a sudden, you can scale an analysis where the human, just finds the tumors, and that eventually will get automated, too, but it's a much harder task. And then the AI model basically measuring those tumors across all the scans that have been acquired on a patient during the course of treatment, and from those, extracting these more informative values that tell you more about whether the drug is working or not and let a drug developer make better decisions early in the course of development so that you don't have these expensive late-stage failures.
So that was probably way too long an answer, but that is essentially where things are being used now, essentially in workflow management and diagnosis, and where they are moving towards, at least in the drug development sense, of creating novel biomarkers and creating measurements that are better measurements of drug effects than we currently have.
When better-- fundamentally, the reason we make measurements is to make decisions, and better measurements lead to better decisions. And AI lets us make better measurements.
ALEX MAIERSPERGER: It's the perfect-length answer, and you've already described so many ways that AI is working. I assume that there's some disparity in who has access to the research or how every patient gets this level of detail or a digital twin and all of the research that goes into it. How do we scale this across all disease types? And how does every patient that goes into a doctor know that they're getting the best technology, the best, latest research all pinpointed exactly to their individual cellular level of detail?
GREG GOLDMACHER: Ha. Well, so-- I mean, just from the radiology point of view, I will tell you that we don't get to the cellular level of detail. What we're seeing is indirect downstream measurements or reflections of what's happening at the cellular level. But more broadly what you're asking is about making these AI tools work across diseases and across patients and across settings.
There's a few things here. First of all, I mean, as far as disease types, I think we're probably farthest ahead in cancer because image-based endpoints, radiology-based measurements of treatment effect are really common in oncology. In oncology trials, there are decades of accumulated data where scans have been collected in a systematic way during treatment, after treatment. And therefore, there's just-- and AI is only as good as the training data, and there's just a lot more training data in oncology because of that.
But other therapeutic areas are definitely making progress and also using artificial intelligence tools to do the same kinds of analysis that I've described in oncology.
From the point of view of developing the tools, I mean, of course there's this vast amount of accumulated data, but there are challenges with doing research on it for a variety of reasons. One is just that data that's collected in the course of clinical trials, those are-- the patients have consented to specific uses of that data. And so if data was acquired, but the patients haven't consented to its use in research, then that's a limitation. And obviously, there's a lot of ethical questions around privacy and patient protection that have to be taken into account.
Beyond that, then-- so let's say, with that out of the way, using the data in training AI essentially has to be done in a way where you keep an eye on selection bias and other issues that plague AI research more generally. Some of the challenges regarding privacy and consent can be used-- can be solved with proper anonymization, and there's a difference between just de-identifying data and fully anonymizing it so that it may still be useful for AI research, but it can't be linked to individual patients.
And in general, just doing this research, you just have to have a clear view of the risks. So from an institutional point of view now within a drug development company, we have to think about basically what risks exist if you open up clinical trial data and re-analyze it and whether it could create confusion or false signals that we want to be careful about.
In general, I would say that one of the things that you could really use AI for-- and this is sort of related to the diagnosis case I talked about. When scans are required, having AI do opportunistic screening-- that is, detection of early disease in studies that are done for some other purpose.
So the distinction here is-- so population screening or cohort screening is something like mammography. You're going to get everybody a mammogram, every woman between whatever age is, you're going to do a mammogram every year, and that's cohort screening. So you're looking for a particular disease.
But what about patients just coming through the emergency department and they get a chest X-ray? If somebody's-- a radiologist, when we look at a scan or when we look at a medical imaging study, we are supposed to look at everything, not just at the thing that the ordering doctor asked about. So they may say, you're looking for pneumonia, but of course, you're looking for lung nodules or other kinds of disease. But humans are humans and people are busy and people miss stuff.
And if you have AI tools that are trained to pick up subtle early signs of disease on scans that are being done for other reasons, there's a real opportunity there for earlier diagnosis. But again, here, the challenge is getting access to the right kinds of data to do the training of those AIs because every eye is limited by its training data.
So if you're going to do opportunistic screening, for example, if you want to train AI for that, what you need is you need longitudinal data sets where you can find patients who had the disease, for example, and then go and look for scans that they might have had in the past to use to train the disease-recognizing models.
So those are some of the challenges for getting-- obviously the industry is developing at a rapid pace, there's been a tremendous amount of investment in it. So I think-- and one nice thing about AI tools, by the way, the other-- so in imaging, some of the cool stuff that's happening also is in molecular imaging. So PET tracers that are engineered to go to particular molecules. The challenge with those is that those things are-- logistically can be difficult to do.
So to use a novel PET tracer may require a radiochemistry lab on-site at a hospital, and not every hospital has that. So like these novel PET tracers, you can do it at Mass General or Gustave Roussy or UCSF or what have you, but It's not easy to do in a community setting.
The cool thing about AI tools is they live on a server somewhere in a cloud. So as long as-- and basically, it's using your routine clinical scans, or regular CT plus a little extra math, and getting a lot more information out of that scan. So I think that that is the real promise of AI-based analysis in radiology.
ALEX MAIERSPERGER: Really exciting. Opportunistic screening, thinking about how to scale it to all patients and all disease states. Incredible insight. It does sound like there's also a need for data transparency. You mentioned some of the challenges there and some of the regulatory issues and things. You've obviously-- you're competing to get drugs to market with other companies. So what's the role of collaboration to really advance science? And is data-sharing even feasible or is this a made-up world that I want to live in?
GREG GOLDMACHER: So that's a great question. I think that-- I work closely with many of my colleagues in-- the imaging leaders and other pharma companies. And I think our general approach from the clinical imaging point of view is that our companies compete on the strength of the drugs that they make.
The rapid, robust, standardized, accurate assessment of treatment effects is something that we should all want. That is a tide that lifts all boats. And so when we come up with-- so standardized approaches to measurement of treatment effect is like everybody wants to be doing things roughly the same way because you don't want to confuse an investigator if they have to apply one set of rules for assessment of efficacy and one trial, and in the same disease but in a different trial, applying a same set of efficacy rules, that's confusing, and it basically creates noise that actually it makes developing new drugs harder for everyone.
From a data-- so from a data transparency point of view, though, of course, companies that acquire data as part of their trials are very protective about it. So there's a few things that can be done. I mean, one is that there is a number of consortia-- the Foundation of the National Institutes of Health, just as an example, had the Vopak Project, which went to a bunch of different pharma companies and got pharma companies to donate data for research in novel analytics. And a lot of the approaches that I was describing earlier, radiomics, growth kinetics, that's coming out of these kind of consortia.
Basically, if you can figure out what the actual risks are of sharing some data, you can engineer your way around those risks. So for example, if what a sponsor is concerned about is that reanalysis of a data set may yield some confusing picture of drug efficacy, well, maybe you share just a subset of data from a trial, or just the control arm from the trial, or whatever. depending on the specific type of AI tool that's being developed, that may be enough.
And so essentially, consortia coming up with ways to lower the risks-- of course, trusted third parties is an important factor here-- with proper data-sharing agreements and data use agreements outlined, I think that that's one promising thing.
The other is a technological solution, and that is so-called federated approaches, or basically approaches-- technological approaches where you have a repository where the owner of the data can put their data, but maintain complete control of it. And on the other hand, the creator-- the developer of an AI tool can put their tool in there, and then the tool can look at the data without ever extracting the data. And the owner of the data can see the effectiveness of the tool without ever seeing the code for the tool or the direct model.
So there's both the collaborative approaches driven by trusted third parties, and technological approaches, which basically allow data-owners and algorithm developers to collaborate even in the absence of perfect trust.
ALEX MAIERSPERGER: So it could be the real world, not just the made-up world. That's exciting.
GREG GOLDMACHER: Right.
ALEX MAIERSPERGER: Your breadth and depth, already the expertise on display, it sounds like we could ask you questions from a very wide lens. How diverse is your role at Merck and how did you end up in it?
GREG GOLDMACHER: So I trained in diagnostic radiology clinically. My background had been in neuroscience, my PhD was in neuroscience, and I ended up doing as a fellow research in neuroimaging at Mass General. And got involved in a little clinical trial for a thrombolytic drug that was being done for acute ischemic-- tested in acute ischemic stroke.
I realized that I really enjoyed clinical research and ended up a couple of years later going to one of the imaging CROs, which is an imaging core lab. They collect scans and do independent analysis in the course of trials.
And then in 2015, I came to Merck. For a time, supported a portion of the oncology imaging portfolio, and then took over oncology imaging, and then all of clinical imaging regardless of therapeutic area. And then last year, we added clinical pathology to the mix because assessing trial outcomes using imaging is a matter of a controlled and rigorous way overseeing the collection of samples and the analysis of the samples, transfer to a central facilities, collection of all of that, and overseeing the process.
Well, whether the sample is a scan or a piece of tissue, a lot of the process considerations are the same, so that's why we integrated clinical imaging and pathology.
And then, of course, after I got to Merck, I realized how many tens of thousands of patients' worth of sequential curated scans we had from our trials, and started advocating that we should be doing analysis on this, and over time, have gathered a group of folks doing research in that.
Along the way-- I mean, from a completely different point of view, I went to business school after I got into industry because I figured I should learn to speak the language of industry. And got an MBA, and then have been working with our business development and licensing folks and our internal venture groups on evaluating opportunities to invest in companies or licensing deals with companies that are developing interesting technologies.
So I work with our internal-- one of our internal venture funds and with our BD&L group in evaluating outside opportunities. And now these days, I'm actually I've added the task of integrating some companies that we acquire into Merck, which is a novel challenge for me.
ALEX MAIERSPERGER: You have a few jobs within Merck. You've got the varied background, including research, even the degree you mentioned in finance. That gives you just a lot of vantage points on funding new startups like you talked about, partnering within the existing ecosystem, seeking out what disease states or technologies that you're going to use to make bigger, better, earlier bets on what comes next. And so what should we know about? What's coming next for all of us?
GREG GOLDMACHER: Well, it's hard for me to talk about specifics of what's coming next because there's a bunch of individual projects, and many of them are really exciting, but it's hard to know what specific path to pursue.
I think in the big picture, what I think has the great-- the greatest potential, the greatest promise, in order to develop these tools in the most effective way, is what you really need is you need a partnership between clinical development groups, clinicians, data science groups internally within organizations, and external opportunities. So you really need to be able to essentially coordinate.
In teams that are-- what you really need is diverse teams, and teams with diverse perspectives and abilities. So what you need is you need people who really understand the problems. Those are the clinicians and the clinical development teams. They understand the problems that need to be solved. Then you've got the data science and IT groups. They understand the tools, but they often don't know how those tools-- how to apply those tools to the problems or what the important problems actually are.
And then you've got the external innovation folks. So for example, either internal venture groups in big pharma or other business groups or M&A-focused teams that also deal with licensing for technology that can help direct-- that can basically help direct resources in the right direction.
So you need people who understand the problems, people who understand the tools, and people who can channel resources. And those need to be talking to each other constantly. Because what otherwise what happens is you get data science groups developing terrific tools, but they don't really solve the problems that need to be solved, and so they're never used.
And so for any particular-- any of those individual areas, I can say, they see this, but they don't see this. So everybody know the whole blind men and the elephant thing. Everybody sees a different part of the problem. and what you need is you need everybody to be talking in order to bring these tools forward and to develop the right tools for the right problems to solve problems for patients.
ALEX MAIERSPERGER: Out of the millions of records in radiology that you talked about, the billions of data points, the multiple roles and hats that you wear, Dr. Goldmacher, thank you so much for spending some time with us here on the Health Pulse Podcast.
GREG GOLDMACHER: Pleasure to be here. Thank you so much, Alex.
ALEX MAIERSPERGER: And to our viewers and listeners, we know you also have infinite ways to spend your time. Thank you for spending a little bit of it with us. If you'd like to join as a guest or join in the comments, please send us an email, thehealthpulsepodcast@sas.com. We're rooting for you always.
Creators and Guests
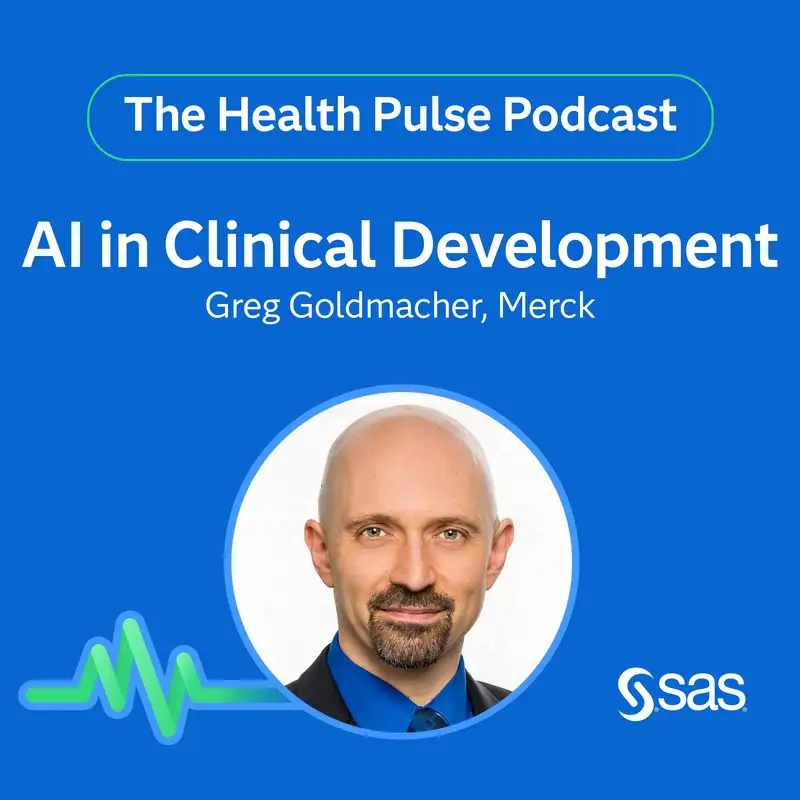