From Research to Patient Care: Bridging the Gap
MARC BERGER: In a learning health system, as you deliver care, you're creating the data set that you can use to analyze it. And it can be curated on a real-time basis, and you can start using that to inform decision making.
[MUSIC PLAYING]
ALEX MAIERSPERGER: Today, we learn from Dr. Marc Berger, a physician, scientist, and researcher who spent a career building what we now call real-world evidence across the biggest life science organizations on the planet.
[MUSIC PLAYING]
Marc, thank you so much for joining us today.
MARC BERGER: Glad to be here.
ALEX MAIERSPERGER: Let's talk about your career progression. You're a physician, a scientist, a researcher. Is it in that order? And do you lean on one of those expertise more than others?
MARC BERGER: Well, I guess the way to put it, ever since I was a little kid, I wanted to be a physician scientist. And I went the usual route to medical school and then did a postdoc. I was doing basic science research with isolated liver cells. And I was doing research, teaching, and patient care.
But then I wanted to simplify my life. So I left academia, and I moved to industry. And since then, I've been doing clinical research, initially phase 2, 3, and 4 clinical trials. But then the bulk of my years, I've spent doing outcomes research or health services research.
ALEX MAIERSPERGER: I like how you said simplify your life and then went into the hardest thing possible, the big challenges.
MARC BERGER: [CHUCKLES]
ALEX MAIERSPERGER: You've talked about the switch from paper records to electronic health records, which, in many ways and places, is still happening today. Have those electronic health records achieved their promise? And how much further can we take health data?
MARC BERGER: Well, it's a very mixed picture. So, even without talking about the electronic health record, digitized health care information really has been around for a long time, mostly for billing purposes. And even in the early days, when I started in the field of outcomes research, you could do a lot of good research using administrative claims data.
With the advent of the electronic health record, we've now got deeper and richer data. But that data is dirty, and it's sparse. So combining it with claims data has been helpful.
But it's still not designed for research. And it's not designed to be user friendly for physicians or caregivers in terms of how they interact with the record and the patient at the same time. So there's a lot of burnout and barriers with electronic health records.
But the promise is there, and we can do a lot of great research with it. There are some barriers that need to be overcome. Privacy, there is concern about this is only going to be used for laudable purposes and research, not used for marketing purposes.
The technology is not seamless with patient care. It actually gets in the way of delivering patient care. It doesn't fall into making it easier for doctors to deliver care.
And then there are economic disincentives in terms of making the data widely available and mergeable. So there are commercial interests. People who have access to large pieces of data, they want to monetize it. They don't really want to share it.
And interoperability is a major problem. Even within the same health care system, it's hard to have interoperability across different locations and different hospitals. So there are a lot of challenges. But eventually, we're going to get there. And the digitization of all health care information is the foundation of our one day reaching our goal of a learning health care system.
ALEX MAIERSPERGER: Can you tell me more about that learning health system? You said some already incredible things about just the barriers for both the patient, the clinician, the staff member. And so obviously, there's some real challenges.
And there's some of those incentives that you talked about, is each area has their own reason to maybe keep some of the data on their side versus being able to share about that. So tell me about the learning health care system. It sounds like we're still a ways away from a true learning health system?
MARC BERGER: Ah, we're very far away from it. So let's start. In a learning health care system, the data is owned by the patients. It's not owned by anybody who collects it. And the patients would agree that the data could be used for improving care and better tailoring treatments to the right patient, at the right time, at the right dose.
It would also be real time. Right now, research is done in one-off experiments. You do a clinical study, and you report the results. But in a learning health care system, you could have continuous learning going on and do learning by doing, as well as having experiments embedded directly in the health care system. So in a learning health care system, you're not reliant upon, what is the published literature? You know up to the moment, what is the best available information to inform evidence-based decisions to be made jointly by a provider and a patient?
So if you go into a doctor today, and you need to have surgery, you know the reputation of the doctor. You may even know the quality metrics of the hospital he's at. But what you'd really like to know is, how many patients exactly like you are facing the same medical problem? What were their choices of care, and what were their outcomes? And you'd like to have that inform, whether you choose that hospital and that surgeon.
Similarly, most of the information we have today is about the average patient. Our clinical trials talk about the average patient. And it's usually a very select group of patients that don't have intercurrent conditions. So we have the best chance to measure a treatment effect.
But most patients don't have no other conditions. They have other conditions. So what you really want to know is a patient like you who is-- make it up-- 60 years of age, and you have hypertension, and you have pre-diabetes, and you used to smoke, but you don't smoke anymore. What are the outcomes in patients like those? And can we have that inform the choices that you and your physician make together?
And in a learning health care system, this will be done seamlessly. Because the information will be at the doctor, at the provider's, fingertips. And it will be used to not only make decisions at the bedside, but better design health benefits, better design support activities like patient care management or disease management. It will make the whole system hum.
Right now, even in countries with a single-payer system, they're having enormous difficulty in delivering care in an efficient manner to all patients that need it. And certainly in the US, we don't have a health care system. We have a fragmented mess. And people are very frustrated trying to navigate through their insurance and how they can make sure they're going to get the right care that would be best for them.
So we have a long way to go. But ultimately, with a combination of public and private investment, we can eventually reach a place where the doctor won't need to be typing a thing into a electronic health record. The interaction will automatically be recorded, and it will be coded into data.
And the record will automatically fill in any experience that you've had with the health care system before or any other information that you've decided to provide the health care system. And it would be seamlessly put together, and the decision support would be there to help doctors make better decisions. But we're a long ways away.
ALEX MAIERSPERGER: It sounds like one of the real enablers of that and opportunities is that combination of research data or data used for research and commercial purposes and care delivery, that combination. You're one of the pioneers of health data marts. Is that the early stages of real-world evidence and bringing that together?
Now that we have advances in AI and machine learning, where does that get us current day? It sounds like you said, we're still far from there. But it's been a journey to get where we are, right?
MARC BERGER: Absolutely. So back when I started in clinical research and outcomes research in the '90s, as I said, we had access to administrative claims data. And that was about it, and some survey data. And then starting sometime in this century or millennium, we started to get access to electronic health record data. And those data proved incredibly fruitful to help us see what was working in actual patients as opposed to what could work.
So clinical trials show you what could work under ideal circumstances. But you want to know, what does work when ordinary physicians are taking care of patients who may not always take all the medication that you give them or may not always understand all the instructions? And so that's been terrific.
And so I built a data mart at Merck in 1995. I built a data mart at Lilly in 2007. I built a data mart at Pfizer, 2012, I think.
But all of these are one-offs and allowed data scientists and researchers to access the data and better refine questions that they want to do in terms of drug development. But that's not the same as removing the distinction between research and patient care.
In a learning health care system, as you deliver care, you're creating the data set that you can use to analyze it. And it can be curated on a real-time basis. And you can start using that to inform decision making.
So the separation of research from clinical care, that's very much a 20th century paradigm. We've moved closer. But at some point in the future, it's going to be seamlessly one. And that will be an amazing time.
ALEX MAIERSPERGER: On the real-world evidence side, the watches and wearables and some of the sensors that we have now or some of the self-reported data, what type of advancements need to happen in that? Is it a collection challenge? Is it a patient recruitment challenge? Is it something in the data or something in the-- the sensors aren't small enough yet? How much further do we have to go to get to that real patient outcome reporting?
MARC BERGER: Well, we've made enormous progress. I mean, we can continuously monitor people's blood glucose levels. But we really don't know what to do with all that data. So most of the research has been done on periodic sampling of blood glucose data.
How to maximize the use of continuous data? We're not sure how to use that. And then other kinds of information that people provide, whether it's on a website, where they talk about how much problem they're having to walk or how much trouble they have walking upstairs, we don't know how to integrate that and validate that data.
So a lot of data collected by sensors is really interesting. But the problem is, even with those data, patients don't wear the sensors all the time. So if-- a lot of people are monitoring sleep, and then they suddenly get no data from it, is it because the patient's not slept? Or is it because he just took the monitor off?
So there are lots of challenges here. And ultimately, with all kinds of real-world information, all real-world data, we have to develop quality standards. So that we know that when we interrogate that data, it is fit for purpose. In other words, it has the ability, the accuracy, and the relevance, and the reliability to be able to answer questions in a robust way.
Because if you're using bad data, then it's the old saying, garbage in, garbage out. So we're a long way from establishing universal standards for all the different kinds of real-world data. But most of the attention right now is focusing on trying to develop consensus standards around electronic health record data, which is appropriate. But there's still a lot of work to be done.
ALEX MAIERSPERGER: Incredible lessons in the past, present, and future here. So we're going to jump into some of the hard-hitting speed round questions. People always love to get a little taste of outside of work life. And so we'll hit a few fast-paced questions here. And then we'll jump back into the future of quantum computing and AI, machine learning, where everything's going to take us in the future. But the people want to know, what's your favorite dessert?
MARC BERGER: Ice cream.
ALEX MAIERSPERGER: Flavor? Or just equal opportunity ice cream?
MARC BERGER: Well, I love coffee chocolate chip or coffee toffee crunch.
ALEX MAIERSPERGER: One place on your travel bucket list.
MARC BERGER: Well, I've traveled an awful lot. So I've visited all seven continents. I've hiked the Himalayas. I've camped out in the Sahara desert. I've seen the Northern Lights, and I've seen the Southern Cross. So most of my travel these days is to visit my grandchildren. But one place I do want to go, I haven't been to La Scala, in Milan. I'd like to go to an opera at La Scala.
ALEX MAIERSPERGER: It sounds like we could do a whole show on these seven continents. We could ask about all your travels. What's your go-to workout or exercise?
MARC BERGER: I'm not a big exercise person, but I do take frequent three-mile walks. And there's lots. I live in Manhattan, and there's just lots of places to walk that are just fun to walk around.
ALEX MAIERSPERGER: What's your favorite app on your phone?
MARC BERGER: Hmm, I don't know if I have a favorite app. I guess the one I use most is Gmail.
ALEX MAIERSPERGER: Physical books or movies?
MARC BERGER: Both. So I really got into film when I was in college. I had some great film courses, where I was introduced to Francois Truffaut and Ingmar Bergman and Akira Kurosawa. And I still love films. And I subscribe to the Criterion Channel, which has a lot of wonderful movies on it.
But I read books. And so right now, I'm reading Eugene Oneguine, by Pushkin. And just before that, I read a biography of Roger Penrose. But the books aren't physical anymore because I don't have room in my small apartment for physical books. So I read them all on Kindle.
ALEX MAIERSPERGER: That's fair, though. How about morning or night?
MARC BERGER: Neither. I like to sleep late, and I like to go to bed early. When I was younger, I was a night owl, not so much anymore.
ALEX MAIERSPERGER: This was so much fun. We share a tremendous love of ice cream and a disdain for exercise. So I feel we're kindred spirits here. Incredible, really appreciate you sharing the inside look at your life here.
We're going to jump back to something that you said that I think is-- I love the idea of how far we've come, but how far we still have to go. And so you talked about AI and machine learning and how in some cases, we have some of the components of the data, or we have new sets of data, or bigger, broader data sets. And we just don't know what to do with it yet, or maybe we can't do it because of compute.
Now the next word is "quantum computing," the next big thing. With quantum computing and potentially AI enabled by quantum, what does that get us? What does that even mean? What sort of timeline are we looking at for progress?
MARC BERGER: So let's back up. So I'm not a big fan of the term "artificial intelligence." A lot of what we call AI is basically, machine learning, which is a data analytic approach. There are many kinds of machine learning that you can do. It's basically turning everything into categorization problems.
And the value of machine learning is that it can handle much larger data sets that have many more features. So most of our data sets today, in health care anyway, if they have 50 or 100 features, that's a lot. When you have 1,000 features or 10,000 features or more, then simple regression modeling can't really deal with that anymore. And that's when you need to start using machine learning, and particularly as we start merging different kinds of data together.
So we get claims with EHR, with patient-reported data, with weather data, whatever else you can imagine. We merge that all. We're going to have to start using more of these machine learning and AI techniques.
The problem with machine learning is that-- well, there are two problems. One is the hardware we have for it is not where we need it to be. So there has been a whole lot of excitement about NVIDIA chips, graphical programming units, which are better than CPUs. And that really has enabled advances in AI. But it's nowhere where it needs to be.
And even if you link together many GPUs, you still can't do what you want to do. And in real machine learning, you want to examine all possible relationships. But we can't do that. So when we do analysis today of genomic data or other kind of data, we select where we're going to look based upon what the literature told us. Because if we try to look everywhere, the runtime would be excruciating long and not doable.
So the promise of quantum computing is that instead of doing a lot more computations, you can do logarithmically more computations, which should make a lot more computations doable in a reasonable period of time. The other problem with artificial intelligence-- and I'm now talking more about generative AI, which everybody is all excited about-- is that generative AI has already been shown to be incredibly powerful for many things.
And Eric Topol, in one of his talks, showed how it can make interpretations of EKGs that have nothing to do with heart disease-- although we don't know how it's making its interpretations, it's correct. But we haven't validated that. And it's one thing to make decisions which are not irrevocable, which you have data that you're not completely sure of. It's another thing to do it when it could be irrevocable.
And we know that artificial intelligence hallucinates, and it's not stable. It's continuously changing itself. So how do we put guardrails around AI and make sure that if we think we're discovering something new, some new truth, we really discovered a new truth?
Now, I'm not worried about its being used-- a lot of commercial uses for AI, chatbots and everything. That's OK. But we need to be very careful about how we use AI. However, you can't hold back the future. And the hardware will improve. AI methodology will improve. And some of the things that we see in science fiction movies will eventually come to place if we don't blow ourselves up.
ALEX MAIERSPERGER: You've built teams ahead of their time, so the data mart and the combining research and clinical data, and pulling in the administrative side. How do you future-proof the workforce? And so it sounds like we're going to need all sorts of both the hardware and the software side and maybe the people skills side to be able to have the conversations, and make the bets, and do the coding. How do you build a team, especially within a life sciences company, that has those talents or attributes that maybe are 10 years out, but you've got to build for that now?
MARC BERGER: Well, I've been building the same team for 35 years, although I'm not-- I'm semi-retired now. But it's a cross-functional team. No one can have all of the knowledge and expertise you need to have. You need to have people who either understand coding or understand data science and are familiar with how things are changing with AI now.
You need to have people who are excellent methodologists. Because doing research with health care data is not an easy task. And you can make a lot of mistakes. So the expertise in epidemiology and outcomes research is incredibly important. And a lot of bad research has been published over the last 30 years, a lot of good stuff, but a lot of bad stuff.
And then you need to have clinical and basic science experts who have the knowledge of what's really going on in patients and what the physiology and biochemistry is, in order to be lucky enough to have insights that are really interesting. And so building that kind of team is hard. Because especially in pharma companies, a lot of this expertise is siloed in separate departments.
And it's hard for them to collaborate. And when they do collaborate, there's always jockeying for position about who's in charge and who's going to get the credit. It's not about getting the credit. It's about asking good questions and finding new insights that will make it easier to develop better and new treatments within a health care company.
But the same is true with any kind of research. The use of AI in research, we need to be careful about how we use it so we're sure we're finding the truth. Now, the data is the data. I don't care what the source of data is. But tracking the raw data that is collected, and then seeing how it is curated and quality controlled, and understanding what happens to it along the way, and then having a track record about how reliable it's been when you've tried to use it to answer questions, these are things that we're just only beginning to approach right now.
So I believe the future will come. I've always found that the future comes more slowly and more rapidly than you think. So the things I've been talking about, about real-world data and data quality and careful approaches to the methodology and analyzing the data, I've been talking about that for more than 30 years. And it's still what everybody's talking about. And pharma still hasn't fully embraced it. And the same is true about the health care system, I think.
ALEX MAIERSPERGER: On the pharma side, being a physician, seeing the research firsthand, being a scientist, where's the biggest value from a quantum computing, AI, machine learning, all the advanced technology? Is it in new drug discovery, and so it will be things that don't exist yet? Is it in drug repurposing? Is it in the personalized medicine, just tailoring dosage and time and place to the right person?
You've mentioned science fiction. Is it we're going to cure cancer at some point fairly shortly? Is it we're going to live till 500? What's the real opportunity?
MARC BERGER: So golly, it's a great question. So data interrogation, whether it's standard statistics or using AI, is very useful all across the life cycle, from discovery through post-marketing. In discovery-- and that's where I think a lot of the impact is going to come with AI in the shorter term-- we are not humble enough about what we don't know.
We know some of the mechanisms of diseases. But diseases are a lot more complicated. And so we think we've unspooled all the secrets of the genome. But we haven't. We know what all the genes are, but the stuff in between is not junk. It's really important. And we're just beginning to learn what it is.
And then there is proteins that attach to the genes, which is even harder to analyze, which is epigenomics, which turn genes on and off. Eventually, eventually, we'll have data sets of incredible size, that the only way to interrogate will be with AI and quantum computing. And when we have longitudinal data sets that look at these changes in all the biochemical parts of what controls the cell with the clinical manifestations over time, we're going to be at a whole new level of understanding disease and have a whole lot of better idea about how to intervene.
But as smart as we are, we need to be humble. This is not going to happen overnight. We're not going to cure all cancer in the next 20 years. I'd love to, but we're not.
But some of the applications right now through combinatorial chemistry can now be done in silico. So you can figure out how molecules go all together. And you know about what active groups different molecules have. So you can do a lot of development that used to be wet chemistry. You can do it now inside the computer and speed that up.
And we have high throughput screening processing that allows us to screen for many more compounds. So there are many things that will be advanced. But right now, still, although we have a much better understanding of discovery, our success rate in developing new treatments is still relatively low. There are still many more failures than successes that come out of biopharmaceutical companies.
ALEX MAIERSPERGER: All the things that you said, so organizational structures, building teams, the advanced technologies, and future technologies, the people and the workforce, what makes you most optimistic that we're going to have a healthier future?
MARC BERGER: Well, by nature, I am an optimist. So I get accused of that all the time. When I think about what medicine was like when I was an intern in 1978 to today, it's wholly different. Or when I was a kid, did I even know there was a thing called computers? I was a teenager when we landed on the moon. But the world changes in ways we can't predict. So, again, as long as we are kinder to each other as people, and we can learn to live together on this planet, I believe that progress is inevitable.
[MUSIC PLAYING]
ALEX MAIERSPERGER: What an incredible conversation. What an incredible amount of learning of past, present, and future. And I love your optimistic take. Marc, thank you so much for being here today.
MARC BERGER: All right. Thank you very much. Have a great day.
ALEX MAIERSPERGER: Thanks for listening. If you have questions, or you want to join as a guest, please email us, TheHealthPulsepodcast@sas.com. See you next time.
[MUSIC PLAYING]
Point at people and the shift to electronic records and all that that got us, all that that got us-- thanks for spending some time with us. Thanks for learning along with us. Or thanks for-- and you, thanks for listening. You stay classy, San Diego. Should we do a take two just in case?
[MUSIC PLAYING]
Creators and Guests
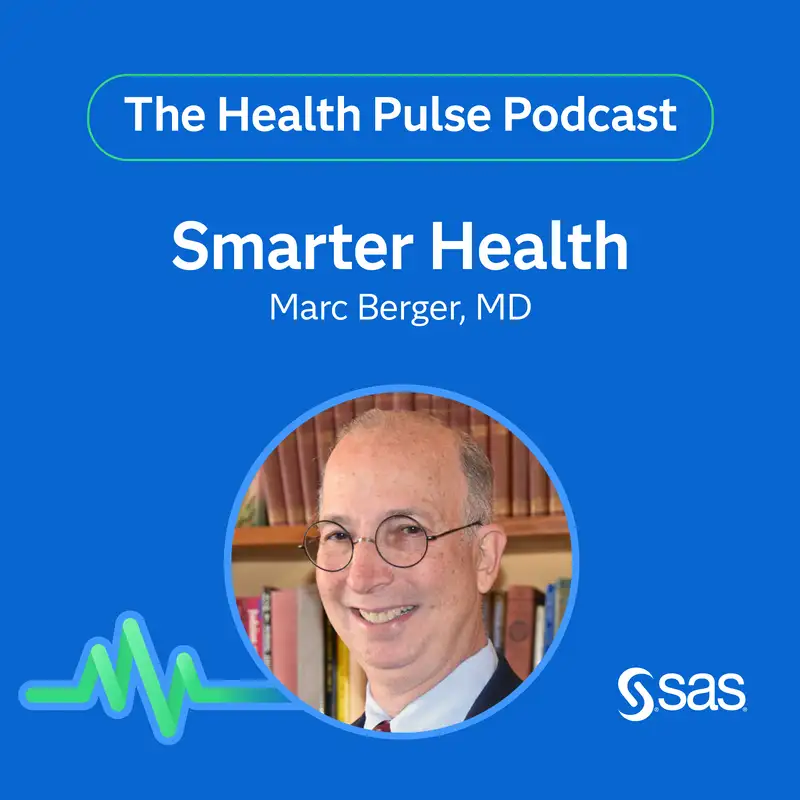